
International Journal For Multidisciplinary Research
E-ISSN: 2582-2160
•
Impact Factor: 9.24
A Widely Indexed Open Access Peer Reviewed Multidisciplinary Bi-monthly Scholarly International Journal
Home
Research Paper
Submit Research Paper
Publication Guidelines
Publication Charges
Upload Documents
Track Status / Pay Fees / Download Publication Certi.
Editors & Reviewers
View All
Join as a Reviewer
Get Membership Certificate
Current Issue
Publication Archive
Conference
Publishing Conf. with IJFMR
Upcoming Conference(s) ↓
WSMCDD-2025
GSMCDD-2025
AIMAR-2025
Conferences Published ↓
ICCE (2025)
RBS:RH-COVID-19 (2023)
ICMRS'23
PIPRDA-2023
Contact Us
Plagiarism is checked by the leading plagiarism checker
Call for Paper
Volume 7 Issue 4
July-August 2025
Indexing Partners

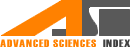
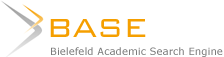



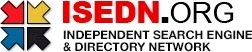

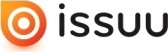

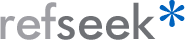
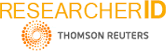
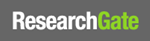
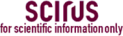
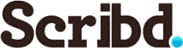


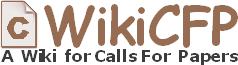

Improving AI Model Performance by Augmenting Synthetic Data
Author(s) | Monojit Banerjee |
---|---|
Country | United States |
Abstract | In recent years, supervised learning has improved many computer vision problems. However, data scarcity, lack of labeled data, and imbalanced datasets have created issues in adopting this improvement in the medical imaging domain. With the recent advancement in other large language and vision language models(eg: chatgpt, DALL-E) generating synthetic data has become easier. However, this is still cost-prohibitive for large-scale datasets specifically image dataset generation. This approach can also may not be suitable for privacy-first datasets. In this work, the proposed methodology is to generate synthetic images based on available labeled images and then use these generated images along with the existing data to solve above mentioned issues. Chest X-ray datasets are one of the complex datasets that suffer from label imbalance problems and strict data privacy is required for handling any such kind of data. In this work, a simplified generative adversarial network-based solution is used which is cost-effective and provides better results than only using available datasets. This proposed method is especially useful for privacy-first, imbalanced datasets. Finally, this solution was compared with some existing proposals. The promising result obtained using this methodology shows that this proposed solution can be expanded to other domains. |
Keywords | Artificial Intelligence, Machine Learning, Synthetic Data, Model training, GAN, MLOps |
Field | Computer > Artificial Intelligence / Simulation / Virtual Reality |
Published In | Volume 6, Issue 2, March-April 2024 |
Published On | 2024-04-30 |
DOI | https://doi.org/10.36948/ijfmr.2024.v06i02.18972 |
Short DOI | https://doi.org/gts4rx |
Share this

E-ISSN 2582-2160

CrossRef DOI is assigned to each research paper published in our journal.
IJFMR DOI prefix is
10.36948/ijfmr
Downloads
All research papers published on this website are licensed under Creative Commons Attribution-ShareAlike 4.0 International License, and all rights belong to their respective authors/researchers.
