
International Journal For Multidisciplinary Research
E-ISSN: 2582-2160
•
Impact Factor: 9.24
A Widely Indexed Open Access Peer Reviewed Multidisciplinary Bi-monthly Scholarly International Journal
Home
Research Paper
Submit Research Paper
Publication Guidelines
Publication Charges
Upload Documents
Track Status / Pay Fees / Download Publication Certi.
Editors & Reviewers
View All
Join as a Reviewer
Get Membership Certificate
Current Issue
Publication Archive
Conference
Publishing Conf. with IJFMR
Upcoming Conference(s) ↓
WSMCDD-2025
GSMCDD-2025
Conferences Published ↓
ICCE (2025)
RBS:RH-COVID-19 (2023)
ICMRS'23
PIPRDA-2023
Contact Us
Plagiarism is checked by the leading plagiarism checker
Call for Paper
Volume 7 Issue 3
May-June 2025
Indexing Partners

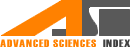
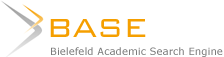



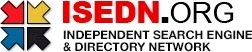

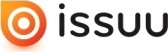

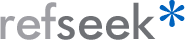
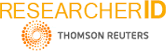
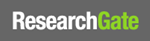
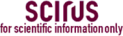
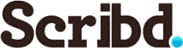


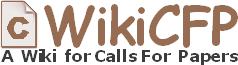

Fine-Tuning Pre-Trained Language Models for Improved Retrieval in RAG Systems for Domain-Specific Use
Author(s) | Syed Arham Akheel |
---|---|
Country | USA |
Abstract | Large Language Models (LLMs) have significantly advanced natural language understanding and generation capabilities, but domain-specific applications often necessitate supplementation with current, external information to mitigate knowledge gaps and reduce hallucinations. Retrieval-Augmented Generation (RAG) has emerged as an effective solution, dynamically integrating up-to-date information through retrieval mechanisms. Fine-tuning pre-trained LLMs with domain-specific data to optimize retrieval queries has become an essential strategy to enhance RAG systems, especially in ensuring the retrieval of highly relevant information from vector databases for response generation. This paper provides a comprehensive review of the literature on the fine-tuning of LLMs to optimize retrieval processes in RAG systems. We discuss advancements such as Query Optimization, Retrieval-Augmented Fine Tuning (RAFT), Retrieval-Augmented Dual Instruction Tuning (RA-DIT), as well as frameworks like RALLE, DPR, and the ensemble of retrieval based and generation-based systems, that enhance the synergy between retrievers and LLMs. |
Keywords | Retrieval-Augmented Generation, Large Language Models, Domain-Specific Fine-Tuning, Information Retrieval, RAFT, RA-DIT |
Field | Computer Applications |
Published In | Volume 6, Issue 5, September-October 2024 |
Published On | 2024-10-22 |
DOI | https://doi.org/10.36948/ijfmr.2024.v06i05.22581 |
Short DOI | https://doi.org/g82hrk |
Share this

E-ISSN 2582-2160

CrossRef DOI is assigned to each research paper published in our journal.
IJFMR DOI prefix is
10.36948/ijfmr
Downloads
All research papers published on this website are licensed under Creative Commons Attribution-ShareAlike 4.0 International License, and all rights belong to their respective authors/researchers.
