
International Journal For Multidisciplinary Research
E-ISSN: 2582-2160
•
Impact Factor: 9.24
A Widely Indexed Open Access Peer Reviewed Multidisciplinary Bi-monthly Scholarly International Journal
Home
Research Paper
Submit Research Paper
Publication Guidelines
Publication Charges
Upload Documents
Track Status / Pay Fees / Download Publication Certi.
Editors & Reviewers
View All
Join as a Reviewer
Get Membership Certificate
Current Issue
Publication Archive
Conference
Publishing Conf. with IJFMR
Upcoming Conference(s) ↓
WSMCDD-2025
GSMCDD-2025
Conferences Published ↓
RBS:RH-COVID-19 (2023)
ICMRS'23
PIPRDA-2023
Contact Us
Plagiarism is checked by the leading plagiarism checker
Call for Paper
Volume 7 Issue 2
March-April 2025
Indexing Partners

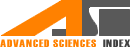
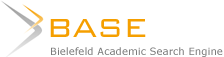



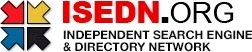

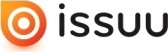

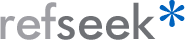
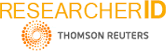
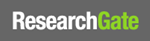
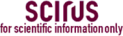
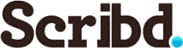


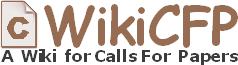

Revolutionizing Cybersecurity with AI and Federated Learning: A Privacy-Preserving Approach for Distributed System
Author(s) | Syed Umer Hasan, FNU Sahil, Soorajj kumar, Ashish Shiwlani |
---|---|
Country | Pakistan |
Abstract | Greater storage, processing, and communication reliance on distributed systems have led to significant cybersecurity issues. Often operating in highly diverse and decentralized environments, these systems conflict with many traditional approaches to security in terms of privacy regulations and user trust. Federated Learning has come as a novel paradigm where it is possible to execute decentralized machine learning by locally training models on data in every device, thus avoiding the transferring of data to a centralized server and ensuring privacy. When FL works in combination with AI, it is highly effective against threats for cybersecurity by enabling timely detection, anomaly identification, and proactive response mechanisms. This work investigates the integration of FL and AI for privacy-centric cybersecurity challenges in distributed systems, its architecture, use cases, and challenges, and the practical implications this approach has on reshaping secure computing paradigms. The findings underline the critical equilibrium between privacy and security while using FL-AI systems for future distributed environments. |
Keywords | Federated Learning, Artificial Intelligence, Privacy-Preserving, Cybersecurity, Distributed Systems, Decentralized Machine Learning, Threat Detection, Data Security, Anomaly Detection, Privacy Regulations. |
Field | Computer > Network / Security |
Published In | Volume 5, Issue 3, May-June 2023 |
Published On | 2023-06-07 |
Share this

E-ISSN 2582-2160

CrossRef DOI is assigned to each research paper published in our journal.
IJFMR DOI prefix is
10.36948/ijfmr
Downloads
All research papers published on this website are licensed under Creative Commons Attribution-ShareAlike 4.0 International License, and all rights belong to their respective authors/researchers.
