
International Journal For Multidisciplinary Research
E-ISSN: 2582-2160
•
Impact Factor: 9.24
A Widely Indexed Open Access Peer Reviewed Multidisciplinary Bi-monthly Scholarly International Journal
Home
Research Paper
Submit Research Paper
Publication Guidelines
Publication Charges
Upload Documents
Track Status / Pay Fees / Download Publication Certi.
Editors & Reviewers
View All
Join as a Reviewer
Get Membership Certificate
Current Issue
Publication Archive
Conference
Publishing Conf. with IJFMR
Upcoming Conference(s) ↓
WSMCDD-2025
GSMCDD-2025
Conferences Published ↓
RBS:RH-COVID-19 (2023)
ICMRS'23
PIPRDA-2023
Contact Us
Plagiarism is checked by the leading plagiarism checker
Call for Paper
Volume 7 Issue 2
March-April 2025
Indexing Partners

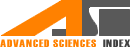
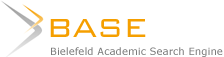



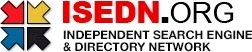

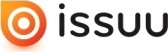

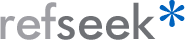
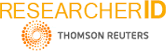
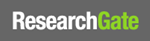
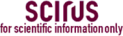
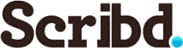


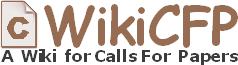

Facial Recognition Using Oculusion Aware Module Network
Author(s) | Srishti Tilkar |
---|---|
Country | India |
Abstract | Facial recognition technology has become a critical tool across various domains, including security, healthcare, and banking, offering reliable methods for identification and verification based on unique facial features. However, several factors can significantly hinder the performance of facial recognition systems, including occlusions (e.g., masks and glasses), pose variations, inconsistencies in illumination, and the effects of aging. These challenges can degrade the accuracy of face recognition systems, particularly in real-world environments where such variations are prevalent. In response to these limitations, this work introduces the Occlusion-Aware Module Network (OAM-Net), a novel and robust framework specifically designed to address the challenges of occluded face recognition. OAM-Net consists of two complementary sub-networks that work synergistically to enhance performance under occlusion. The first sub-network, the occlusion-aware sub-network, incorporates an adaptive attention module that dynamically adjusts the convolutional kernel weights, enabling more effective processing of occluded facial features. The second sub-network, the key-region-aware sub-network, integrates a Spatial Attention Residual Block (SARB) to precisely identify and localize critical facial regions, even when portions of the face are obscured. Additionally, OAM-Net employs a meta-learning-based strategy, which improves the model's ability to generalize across a variety of occlusion scenarios and enhances its overall accuracy. This meta-learning approach enables the model to effectively learn from diverse occlusion conditions and perform reliably in different settings. Experimental evaluations demonstrate that OAM-Net significantly outperforms existing state-of-the-art methods in occluded face recognition tasks. The results underscore the framework’s potential for practical, real-world applications in diverse fields, ranging from security to user authentication. Facial recognition systems, while powerful, face several technical challenges that can compromise their performance. These challenges include occlusions, which obscure key facial features due to the presence of masks, glasses, or other obstructions; variations in illumination and pose, which can distort facial features; and the impact of aging on facial appearance over time. Furthermore, temporal changes and the aging process introduce additional complexity, as long-term recognition becomes increasingly difficult. Moreover, the imbalance in training datasets often leads to biased models, further reducing system accuracy and reliability. Another significant challenge lies in ensuring real-time processing with low latency, which is critical for large-scale applications involving extensive populations. Scalability and generalization across diverse scenarios and environments are also key concerns. In addition, the high computational demands of deep learning models, the risk of over fitting, and the integration of complex components such as attention modules complicate the development process. Finally, privacy concerns and the need to protect facial data from adversarial attacks add layers of complexity to the deployment and ethical use of facial recognition technology. In conclusion, this study presents OAM-Net as a significant advancement in the field of occluded face recognition, offering a robust and effective solution to mitigate the limitations imposed by occlusions, pose variations, and other challenging conditions. The framework's ability to handle diverse occlusion scenarios, improve accuracy, and ensure reliable performance in real-world applications makes it a promising contribution to the future of facial recognition systems. |
Keywords | Facial Recognition , OAM (Oculusion Aware Module Network) , Machine Learning , Deep Learning , Real Time Processing , OpenCV |
Field | Computer > Artificial Intelligence / Simulation / Virtual Reality |
Published In | Volume 7, Issue 1, January-February 2025 |
Published On | 2025-02-23 |
DOI | https://doi.org/10.36948/ijfmr.2025.v07i01.37595 |
Short DOI | https://doi.org/g854h3 |
Share this

E-ISSN 2582-2160

CrossRef DOI is assigned to each research paper published in our journal.
IJFMR DOI prefix is
10.36948/ijfmr
Downloads
All research papers published on this website are licensed under Creative Commons Attribution-ShareAlike 4.0 International License, and all rights belong to their respective authors/researchers.
