
International Journal For Multidisciplinary Research
E-ISSN: 2582-2160
•
Impact Factor: 9.24
A Widely Indexed Open Access Peer Reviewed Multidisciplinary Bi-monthly Scholarly International Journal
Home
Research Paper
Submit Research Paper
Publication Guidelines
Publication Charges
Upload Documents
Track Status / Pay Fees / Download Publication Certi.
Editors & Reviewers
View All
Join as a Reviewer
Get Membership Certificate
Current Issue
Publication Archive
Conference
Publishing Conf. with IJFMR
Upcoming Conference(s) ↓
WSMCDD-2025
GSMCDD-2025
Conferences Published ↓
RBS:RH-COVID-19 (2023)
ICMRS'23
PIPRDA-2023
Contact Us
Plagiarism is checked by the leading plagiarism checker
Call for Paper
Volume 7 Issue 2
March-April 2025
Indexing Partners

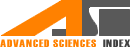
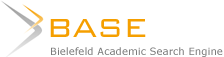



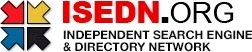

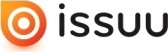

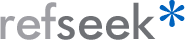
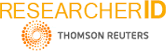
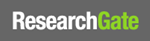
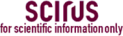
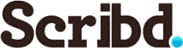


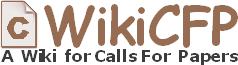

Anomaly Detection for Network Traffic Using Machine Learning
Author(s) | Rathesh Prabu S.S, Jagan Santhosh Kumar J, Sonia Jenifer Rayen |
---|---|
Country | India |
Abstract | It is found that advanced persistent cyber threats transcend the capability of the traditional network security systems in accurately identifying and preventing threats. To help tackle this, anomaly detection has risen to spotlight as a way of identifying strange network activity, which would signify the existence of malware. This work concerns the design of an enhanced network anomaly detection system based machine learning; the work uses the Random Forest algorithm. The advantages of the proposed system have been labeled as ‘signals of value’ which include the ability to analyze flow anomalies such as DoS, unauthorized access attempts, data exfiltration and other malicious activities in Network Traffic for further improvement of Network Security. These features of the network detail includes the packet size, packet protocol type and communication pattern which the system uses to train its model for accurate data results. The performance of the system was tested in a number of experiments that proved very high accuracy levels, precision and recall rates, thus proving that the proposed system can indeed be effective in real-time detection applications. The model was also superior to FW+AVG because it provided generalization to new attacks and the ability to minimize the false positives. Fur- thermore, the system accomplishes its functionalities effectively in dynamic network conditions and cautions appropriately to strengthen the network administration against potential threats. The outcomes of this study add values to the current literature on network anomaly detection leading to the provision of directions for further enhancement of the research, including the integration of learning from other techniques in real-time and addressing class imbalance. This paper insinuates that the future of network security is very bright because organizations can reduce the risk posed by incipient cyber threats by employing machine learning anomaly detection |
Keywords | Network Anomaly Detection, Machine Learning, Random Forest, Cybersecurity, Network Security, Real-Time De- tection, Anomaly Detection System, Intrusion Detection, Network Traffic, Feature Engineering, Class Imbalance, Denial-of-Service, Unauthorized Access, Attack Detection, Network Defense. |
Field | Computer > Artificial Intelligence / Simulation / Virtual Reality |
Published In | Volume 7, Issue 1, January-February 2025 |
Published On | 2025-02-28 |
DOI | https://doi.org/10.36948/ijfmr.2025.v07i01.37761 |
Short DOI | https://doi.org/g86w7s |
Share this

E-ISSN 2582-2160

CrossRef DOI is assigned to each research paper published in our journal.
IJFMR DOI prefix is
10.36948/ijfmr
Downloads
All research papers published on this website are licensed under Creative Commons Attribution-ShareAlike 4.0 International License, and all rights belong to their respective authors/researchers.
