
International Journal For Multidisciplinary Research
E-ISSN: 2582-2160
•
Impact Factor: 9.24
A Widely Indexed Open Access Peer Reviewed Multidisciplinary Bi-monthly Scholarly International Journal
Home
Research Paper
Submit Research Paper
Publication Guidelines
Publication Charges
Upload Documents
Track Status / Pay Fees / Download Publication Certi.
Editors & Reviewers
View All
Join as a Reviewer
Get Membership Certificate
Current Issue
Publication Archive
Conference
Publishing Conf. with IJFMR
Upcoming Conference(s) ↓
WSMCDD-2025
GSMCDD-2025
Conferences Published ↓
RBS:RH-COVID-19 (2023)
ICMRS'23
PIPRDA-2023
Contact Us
Plagiarism is checked by the leading plagiarism checker
Call for Paper
Volume 7 Issue 2
March-April 2025
Indexing Partners

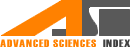
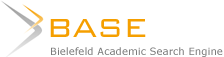



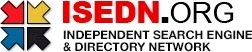

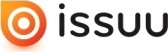

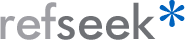
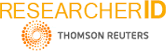
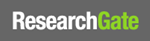
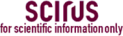
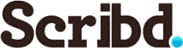


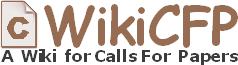

The Role of Quantum Neural Networks in Fraud Detection: Opportunities and Challenges
Author(s) | Anish Naidu Basa |
---|---|
Country | United States |
Abstract | Financial fraud is a growing concern across global markets, and its complexity continues to increase with the expansion of digital banking, e-commerce, and online payment platforms. Traditional artificial intelligence (AI) techniques, such as Logistic Regression and Random Forest Classifiers, have been widely employed to detect fraudulent patterns in massive datasets. However, these classical AI models often grapple with scalability, high computational costs, and difficulties in adapting to rapidly evolving fraud tactics. To address these challenges, the emerging field of Quantum Machine Learning (QML) proposes the integration of quantum computing principles into AI algorithms. In particular, Quantum Neural Networks (QNNs) show promise in drastically improving speed and accuracy for fraud detection tasks. This research paper investigates the current state of both classical AI and quantum AI approaches for financial fraud detection and critically analyzes their performance, efficiency, and practicality. By reviewing cutting-edge studies, including those on Variational Quantum Classifiers (VQCs), Quantum Support Vector Classifiers (QSVCs), and Quantum Graph Neural Networks (QGNNs), this paper illustrates how quantum models can potentially achieve higher accuracy and faster processing times, especially when dealing with unbalanced datasets that are commonplace in fraud detection. Hybrid quantum-classical frameworks, such as Quantum Federated Neural Networks, are highlighted as an intermediary solution that merges the advantages of quantum algorithms with the established robustness of classical methods. The findings suggest that while Quantum Machine Learning holds significant promise for detecting fraud in real time, several hardware-related limitations—including quantum error rates and gate fidelity constraints—remain major obstacles. Consequently, research must continue to develop error mitigation strategies and more sophisticated quantum circuits to fully realize the potential benefits of QNNs. Ultimately, this research emphasizes the importance of ongoing studies that bridge theoretical innovations with practical applications, so that quantum AI can evolve from a nascent technology into a transformative force in financial fraud detection and related domains. By offering a clear analysis of the state-of-the-art and proposing future directions, this paper aims to guide researchers, financial institutions, and policy makers toward building more secure and efficient fraud detection systems. |
Keywords | Financial Fraud Detection Quantum Neural Networks (QNNs) Quantum Machine Learning (QML) Classical AI in Fraud Detection Hybrid Quantum-Classical Models |
Published In | Volume 7, Issue 1, January-February 2025 |
Published On | 2025-02-28 |
DOI | https://doi.org/10.36948/ijfmr.2025.v07i01.37949 |
Short DOI | https://doi.org/g86w5m |
Share this

E-ISSN 2582-2160

CrossRef DOI is assigned to each research paper published in our journal.
IJFMR DOI prefix is
10.36948/ijfmr
Downloads
All research papers published on this website are licensed under Creative Commons Attribution-ShareAlike 4.0 International License, and all rights belong to their respective authors/researchers.
