
International Journal For Multidisciplinary Research
E-ISSN: 2582-2160
•
Impact Factor: 9.24
A Widely Indexed Open Access Peer Reviewed Multidisciplinary Bi-monthly Scholarly International Journal
Home
Research Paper
Submit Research Paper
Publication Guidelines
Publication Charges
Upload Documents
Track Status / Pay Fees / Download Publication Certi.
Editors & Reviewers
View All
Join as a Reviewer
Get Membership Certificate
Current Issue
Publication Archive
Conference
Publishing Conf. with IJFMR
Upcoming Conference(s) ↓
WSMCDD-2025
GSMCDD-2025
Conferences Published ↓
RBS:RH-COVID-19 (2023)
ICMRS'23
PIPRDA-2023
Contact Us
Plagiarism is checked by the leading plagiarism checker
Call for Paper
Volume 7 Issue 2
March-April 2025
Indexing Partners

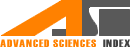
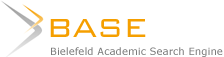



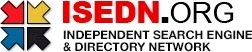

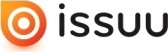

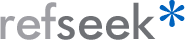
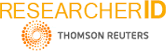
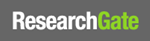
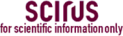
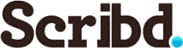


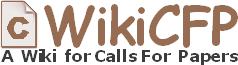

Transformer-Based Deep Learning for Optimized Vehicular Engine Health Monitoring
Author(s) | Durgaprasad Dondapati, Harsha Vardhan Gottipati, Yesu Babu Chattu, Jaya Krishna Aripirala, Thrilochana Devi K |
---|---|
Country | India |
Abstract | The growing reliance on industrial machinery and critical infrastructure has increased the need for real-time, accurate engine health monitoring to prevent failures and optimize performance. Traditional fault detection methods often rely on rule-based systems or shallow machine learning models, which may fail to capture complex patterns in engine behavior. In this study, we suggest a combination of Long Short-Term Memory (LSTM) and Temporal Fusion Transformer (TFT) model for engine health classification. The LSTM captures sequential dependencies in sensor data, while the TFT enhances interpretability by focusing on multi-horizon forecasting and attention-based feature selection. The system is trained on a dataset of engine parameters, preprocessed using normalization techniques, and optimized with a real-time adaptive retraining mechanism to improve model robustness over time. Experimental results demonstrate that our hybrid model achieves high accuracy, outperforming conventional machine learning approaches. Additionally, we integrate our model into a Flask-based web application to enable real-time monitoring and user-friendly fault detection. This research contributes to the advancement of intelligent predictive maintenance, reducing operational costs and enhancing industrial safety. |
Keywords | Engine Health Monitoring, Long Short-Term Memory (LSTM), Temporal Fusion Transformer (TFT), Time-Series Forecasting, Predictive Maintenance, Sequential Data Modeling. |
Field | Computer > Artificial Intelligence / Simulation / Virtual Reality |
Published In | Volume 7, Issue 2, March-April 2025 |
Published On | 2025-04-14 |
DOI | https://doi.org/10.36948/ijfmr.2025.v07i02.39675 |
Short DOI | https://doi.org/g9fm5b |
Share this

E-ISSN 2582-2160

CrossRef DOI is assigned to each research paper published in our journal.
IJFMR DOI prefix is
10.36948/ijfmr
Downloads
All research papers published on this website are licensed under Creative Commons Attribution-ShareAlike 4.0 International License, and all rights belong to their respective authors/researchers.
