
International Journal For Multidisciplinary Research
E-ISSN: 2582-2160
•
Impact Factor: 9.24
A Widely Indexed Open Access Peer Reviewed Multidisciplinary Bi-monthly Scholarly International Journal
Home
Research Paper
Submit Research Paper
Publication Guidelines
Publication Charges
Upload Documents
Track Status / Pay Fees / Download Publication Certi.
Editors & Reviewers
View All
Join as a Reviewer
Get Membership Certificate
Current Issue
Publication Archive
Conference
Publishing Conf. with IJFMR
Upcoming Conference(s) ↓
WSMCDD-2025
GSMCDD-2025
Conferences Published ↓
RBS:RH-COVID-19 (2023)
ICMRS'23
PIPRDA-2023
Contact Us
Plagiarism is checked by the leading plagiarism checker
Call for Paper
Volume 7 Issue 3
May-June 2025
Indexing Partners

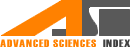
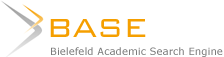



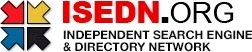

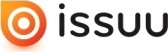

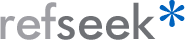
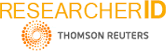
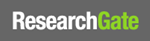
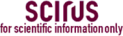
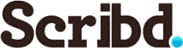


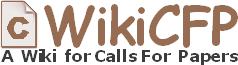

HYBRID DEEP LEARNING MODELS FOR BRAIN TUMOR DETECTION AND SEVERITY ASSESSMENT
Author(s) | Dr. Ms. A Nithya Rani, Ms. JIJI K KARUNAKARAN |
---|---|
Country | India |
Abstract | Abstract— Brain tumor detection is crucial in medical imaging, significantly impacting treatment planning and patient outcomes. Traditional methods often struggle with the complex and heterogeneous nature of brain tumors. This paper introduces a novel hybrid deep learning approach, combining U-Net, ResNet- 101, Generative Adversarial Networks, and Vision Transformers to enhance diagnostic accuracy, model generalization, improve the detection and severity classification of brain tumors. By integrating these state-of-the-art models, the system aims to provide precise tumor segmentation, robust feature extraction, enhanced generalization through data augmentation, and a comprehensive severity assessment, addressing key challenges in brain tumor imaging. U-Net is employed for precise tumor segmentation, while ResNet-101 provides deep feature extraction. GANs augment the dataset, improving robustness and generalization, and ViT captures long-range dependencies in images. These models are integrated using an ensemble learning approach, optimized through a genetic algorithm to assign optimal weights to each model's predictions. The ensemble model effectively captures both global and local features, significantly improving classification accuracy, precision, recall, and score compared to individual models |
Keywords | Brain Tumor, U-Net, ResNet-101, Generative Adversarial Networks |
Field | Computer Applications |
Published In | Volume 7, Issue 2, March-April 2025 |
Published On | 2025-04-29 |
DOI | https://doi.org/10.36948/ijfmr.2025.v07i02.40293 |
Short DOI | https://doi.org/g9g74m |
Share this

E-ISSN 2582-2160

CrossRef DOI is assigned to each research paper published in our journal.
IJFMR DOI prefix is
10.36948/ijfmr
Downloads
All research papers published on this website are licensed under Creative Commons Attribution-ShareAlike 4.0 International License, and all rights belong to their respective authors/researchers.
