
International Journal For Multidisciplinary Research
E-ISSN: 2582-2160
•
Impact Factor: 9.24
A Widely Indexed Open Access Peer Reviewed Multidisciplinary Bi-monthly Scholarly International Journal
Home
Research Paper
Submit Research Paper
Publication Guidelines
Publication Charges
Upload Documents
Track Status / Pay Fees / Download Publication Certi.
Editors & Reviewers
View All
Join as a Reviewer
Get Membership Certificate
Current Issue
Publication Archive
Conference
Publishing Conf. with IJFMR
Upcoming Conference(s) ↓
WSMCDD-2025
GSMCDD-2025
Conferences Published ↓
ICCE (2025)
RBS:RH-COVID-19 (2023)
ICMRS'23
PIPRDA-2023
Contact Us
Plagiarism is checked by the leading plagiarism checker
Call for Paper
Volume 7 Issue 3
May-June 2025
Indexing Partners

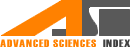
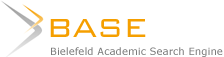



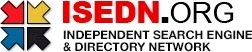

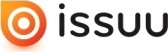

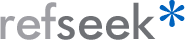
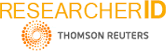
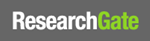
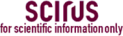
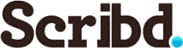


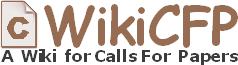

IoT-Based Air Quality Monitoring and Prediction System
Author(s) | Kadagala Venkatesh, Konijeti Venkata Siva Jaswanth, Inavoli Preethi, Jujjavarapu Sri Sai Ganesh, Mandapati Lakshmi Thirupathamma |
---|---|
Country | India |
Abstract | Air pollution is a major environmental issue affecting public health and urban sustainability. Traditional air quality monitoring systems are costly, have limited coverage, and lack predictive capabilities. This paper proposes an IoT-enabled Air Quality Monitoring and Predicting System consisting of MQ-series gas sensors, Raspberry Pi, Random Forest machine learning algorithm, and cloud computing for real-time monitoring and AQI prediction.The system uses MQ-2, MQ-4, MQ-7, MQ-9, and MQ-135 sensors in conjunction with a DHT-11 temperature and humidity sensor, connected to a Raspberry Pi via an MCP3208 ADC. The sensor data is processed using the Random Forest algorithm to provide predictions for AQI. The data is streamed over the ThingSpeak IoT cloud, graphed, and made accessible via a mobile application. Users receive real-time alerts when air quality is poor.Outcomes show that the system gives a sensor accuracy of 92-95 percent and AQI forecast accuracy of 85-90 percent. The cloud-to-mobile latency is approximately three seconds, which means almost instantaneous updates. Compared to conventional monitoring mediums, the system is cost-effective, scalable, efficient, and suitable for use in smart cities and industries.The study concludes that the convergence of IoT, machine learning, and cloud computing makes real-time air quality monitoring and forecasting possible. Some future enhancements include deep learning, edge AI, and increased sensor coverage. |
Keywords | Air Quality Monitoring, IoT, Machine Learning, Random Forest, Raspberry Pi, AQI Prediction, Cloud Computing. |
Field | Engineering |
Published In | Volume 7, Issue 2, March-April 2025 |
Published On | 2025-04-01 |
DOI | https://doi.org/10.36948/ijfmr.2025.v07i02.40370 |
Short DOI | https://doi.org/g9dg2b |
Share this

E-ISSN 2582-2160

CrossRef DOI is assigned to each research paper published in our journal.
IJFMR DOI prefix is
10.36948/ijfmr
Downloads
All research papers published on this website are licensed under Creative Commons Attribution-ShareAlike 4.0 International License, and all rights belong to their respective authors/researchers.
