
International Journal For Multidisciplinary Research
E-ISSN: 2582-2160
•
Impact Factor: 9.24
A Widely Indexed Open Access Peer Reviewed Multidisciplinary Bi-monthly Scholarly International Journal
Home
Research Paper
Submit Research Paper
Publication Guidelines
Publication Charges
Upload Documents
Track Status / Pay Fees / Download Publication Certi.
Editors & Reviewers
View All
Join as a Reviewer
Get Membership Certificate
Current Issue
Publication Archive
Conference
Publishing Conf. with IJFMR
Upcoming Conference(s) ↓
WSMCDD-2025
GSMCDD-2025
Conferences Published ↓
RBS:RH-COVID-19 (2023)
ICMRS'23
PIPRDA-2023
Contact Us
Plagiarism is checked by the leading plagiarism checker
Call for Paper
Volume 7 Issue 2
March-April 2025
Indexing Partners

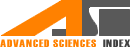
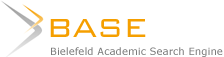



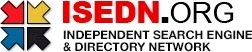

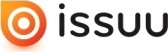

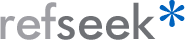
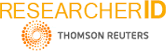
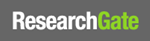
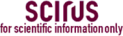
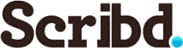


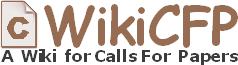

From Pixels to Words: A Deep Learning Approach to Image Captioning
Author(s) | Ms. Isha Panchal, Dr. Jalpa Shah |
---|---|
Country | India |
Abstract | Image captioning, a crucial task in computer vision and natural language processing (NLP), aims to generate meaningful textual descriptions for images. Traditional models use an encoder-decoder framework, where convolutional neural networks (CNNs) extract image features, and sequence models generate captions. However, conventional CNN-based approaches often lack efficiency in feature extraction. To address this, we propose a novel image captioning model integrating EfficientNetB0 as the feature extractor with a Transformer-based encoder-decoder architecture. The Transformer-Encoder, equipped with Multi-Head Attention, refines image feature representations by capturing both global and local dependencies. The Transformer-Decoder consists of two self-attention layers: Self-Attention_1 focuses on previously generated words, ensuring linguistic coherence, while Self-Attention_2 dynamically attends to the refined image features, enabling the model to emphasize relevant visual details at each decoding step. Additionally, an adaptive attention mechanism further optimizes image feature utilization for caption generation. We evaluate our model on the Flickr 8k dataset, demonstrating superior performance. Our results highlight the effectiveness of combining EfficientNetB0 with a Transformer-based encoder-decoder model, achieving improved caption accuracy while maintaining computational efficiency. |
Keywords | Image Captioning, CNN, EfficientNetB0, Deep Learning, Transformer, Multi-Head Attention, Self-Attention, Feature Extraction, Flickr 8k Dataset |
Field | Computer > Artificial Intelligence / Simulation / Virtual Reality |
Published In | Volume 7, Issue 2, March-April 2025 |
Published On | 2025-04-07 |
DOI | https://doi.org/10.36948/ijfmr.2025.v07i02.40378 |
Short DOI | https://doi.org/g9dnbt |
Share this

E-ISSN 2582-2160

CrossRef DOI is assigned to each research paper published in our journal.
IJFMR DOI prefix is
10.36948/ijfmr
Downloads
All research papers published on this website are licensed under Creative Commons Attribution-ShareAlike 4.0 International License, and all rights belong to their respective authors/researchers.
