
International Journal For Multidisciplinary Research
E-ISSN: 2582-2160
•
Impact Factor: 9.24
A Widely Indexed Open Access Peer Reviewed Multidisciplinary Bi-monthly Scholarly International Journal
Home
Research Paper
Submit Research Paper
Publication Guidelines
Publication Charges
Upload Documents
Track Status / Pay Fees / Download Publication Certi.
Editors & Reviewers
View All
Join as a Reviewer
Get Membership Certificate
Current Issue
Publication Archive
Conference
Publishing Conf. with IJFMR
Upcoming Conference(s) ↓
WSMCDD-2025
GSMCDD-2025
Conferences Published ↓
ICCE (2025)
RBS:RH-COVID-19 (2023)
ICMRS'23
PIPRDA-2023
Contact Us
Plagiarism is checked by the leading plagiarism checker
Call for Paper
Volume 7 Issue 3
May-June 2025
Indexing Partners

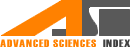
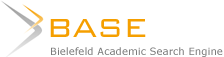



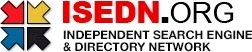

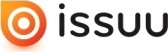

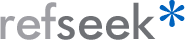
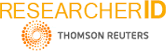
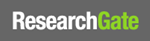
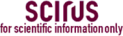
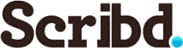


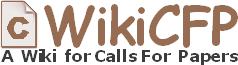

Deep Learning for COVID-19 Prediction and Lung Infection Segmentation from CT Images
Author(s) | Prof. Ms. Yogita Babaji Kajabe, Prof. Dr. Mr. Brijendra Gupta |
---|---|
Country | India |
Abstract | Lung disorders like pneumonia, cancer, and COVID-19, a common and potentially deadly respiratory infection, are very challenging to identify accurately and promptly. Using advances in machine learning (ML) techniques, this research aims to produce a reliable and efficient pneumonia detection system. Chest CT images are used to build a convolutional neural network (CNN) model to differentiate between lung pictures with pneumonia and those that are healthy. Training and validation datasets are a diverse collection of chest CT images collected from multiple sources. With strong sensitivity and accuracy, the proposed approach detects pneumonia with promising results. COVID-19 can also cause long-term harm to the lungs and other organs. When an infected individual coughs, sneezes, or exhales, droplets are released that are the main way the COVID-19 virus is transmitted. Being too heavy to float in midair, these drops quickly land on floors or other objects. The corona virus disease 2019 (COVID-19) started to spread globally in early 2020, as everyone is aware. causing a global health crisis associated with existential issues. Thus, automating the diagnosis of lung infections from computed tomography (CT) images presents a significant possibility to improve the traditional healthcare approach to COVID-19. Nonetheless, there are several challenges in distinguishing infected regions from CT slices, including the broad variety of infection characteristics and the poor contrast between healthy tissues and infections. Moreover, collecting large amounts of data in a short period of time is impracticable, which hinders the training of a deep model. Our proposed technique would analyze the CT scan of the lung to determine the affected lung part and the infected region. The technology will assess the intensity of the inflection and help patients take the appropriate response |
Keywords | CT images, lung infections, pneumonia, COVID-19, convolutional neural networks, and machine learning |
Field | Engineering |
Published In | Volume 7, Issue 2, March-April 2025 |
Published On | 2025-04-04 |
DOI | https://doi.org/10.36948/ijfmr.2025.v07i02.40517 |
Short DOI | https://doi.org/g9dg3j |
Share this

E-ISSN 2582-2160

CrossRef DOI is assigned to each research paper published in our journal.
IJFMR DOI prefix is
10.36948/ijfmr
Downloads
All research papers published on this website are licensed under Creative Commons Attribution-ShareAlike 4.0 International License, and all rights belong to their respective authors/researchers.
