
International Journal For Multidisciplinary Research
E-ISSN: 2582-2160
•
Impact Factor: 9.24
A Widely Indexed Open Access Peer Reviewed Multidisciplinary Bi-monthly Scholarly International Journal
Home
Research Paper
Submit Research Paper
Publication Guidelines
Publication Charges
Upload Documents
Track Status / Pay Fees / Download Publication Certi.
Editors & Reviewers
View All
Join as a Reviewer
Get Membership Certificate
Current Issue
Publication Archive
Conference
Publishing Conf. with IJFMR
Upcoming Conference(s) ↓
WSMCDD-2025
GSMCDD-2025
Conferences Published ↓
RBS:RH-COVID-19 (2023)
ICMRS'23
PIPRDA-2023
Contact Us
Plagiarism is checked by the leading plagiarism checker
Call for Paper
Volume 7 Issue 2
March-April 2025
Indexing Partners

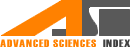
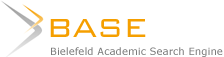



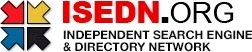

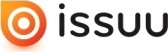

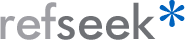
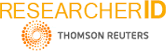
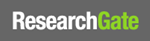
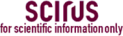
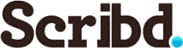


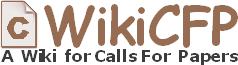

Development Of AI/ML Based Solution For Detection Of Face Swap Based Deepfake Videos
Author(s) | Mr. Chand Riyaj Mulani, Ms. Shweta Dattatray Dugane, Mr. Swarup Jitendra Jadhav, Mr. Siddharth Madhukar Jadhav, Mr. Aditya Mahendra Kamble, Prof. Pradeep Shinde |
---|---|
Country | India |
Abstract | In recent months, free deep learning-based software tools have facilitated the creation of credible face exchanges in videos that leave few traces of manipulation, known as ”DeepFake” (DF) videos. While digital video manipulation has been demonstrated for several decades through the use of visual effects, recent advances in deep learning have significantly increased the realism of fake content and the ease with which it can be created. These AI-synthesized media, popularly referred to as DF, have become widely accessible. Creating DF using artificially intelligent tools is a simple task. However, detecting these DF videos presents a major challenge, as training an algorithm to spot them is not straightforward. We have taken a step forward in detecting DF using Convolutional Neural Networks (CNN) and Recurrent Neural Networks (RNN). Our system utilizes a CNN to extract features at the frame level. These features are then used to train an RNN, which learns to classify whether a video has been manipulated or not. Additionally, it detects temporal inconsistencies between frames introduced by DF creation tools. Our approach consists of a multi-step pipeline. Initially, the input video is processed by extracting frames, followed by facial detection and cropping. The processed frames are then passed to a ResNext CNN model, which extracts deep feature representations of each frame. These feature vectors are subsequently fed into an LSTM-based RNN that captures temporal relationships and inconsistencies between frames. This combination allows our system to effectively differentiate real from manipulated content. To validate our model’s effectiveness, we utilize a diverse dataset comprising videos from sources such as YouTube, FaceForensics++, and the DeepFake Detection Challenge dataset. The dataset is split into 70. Our expected results are evaluated against a large dataset of fake videos collected from standard sources. We demonstrate how our system achieves competitive results in this task using a simple yet effective architecture. The implementation of this system could have significant implications for combating the spread of misinformation, securing digital media integrity, and enhancing trust in online video content. |
Keywords | Deepfakes, faceswap, AI/ML, Detection Depthwise Separable Convolution, Convolution Neural Network (CNN), Social Media, Compressed Video |
Field | Computer > Artificial Intelligence / Simulation / Virtual Reality |
Published In | Volume 7, Issue 2, March-April 2025 |
Published On | 2025-04-05 |
DOI | https://doi.org/10.36948/ijfmr.2025.v07i02.40646 |
Short DOI | https://doi.org/g9dg45 |
Share this

E-ISSN 2582-2160

CrossRef DOI is assigned to each research paper published in our journal.
IJFMR DOI prefix is
10.36948/ijfmr
Downloads
All research papers published on this website are licensed under Creative Commons Attribution-ShareAlike 4.0 International License, and all rights belong to their respective authors/researchers.
