
International Journal For Multidisciplinary Research
E-ISSN: 2582-2160
•
Impact Factor: 9.24
A Widely Indexed Open Access Peer Reviewed Multidisciplinary Bi-monthly Scholarly International Journal
Home
Research Paper
Submit Research Paper
Publication Guidelines
Publication Charges
Upload Documents
Track Status / Pay Fees / Download Publication Certi.
Editors & Reviewers
View All
Join as a Reviewer
Get Membership Certificate
Current Issue
Publication Archive
Conference
Publishing Conf. with IJFMR
Upcoming Conference(s) ↓
WSMCDD-2025
GSMCDD-2025
AIMAR-2025
Conferences Published ↓
ICCE (2025)
RBS:RH-COVID-19 (2023)
ICMRS'23
PIPRDA-2023
Contact Us
Plagiarism is checked by the leading plagiarism checker
Call for Paper
Volume 7 Issue 4
July-August 2025
Indexing Partners

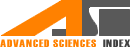
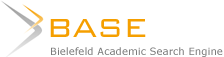



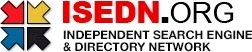

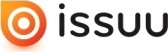

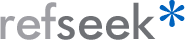
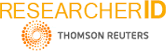
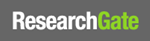
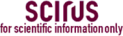
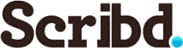


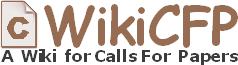

Role of GANs in Simulating Financial Market Behavior for Algorithmic Trading Models
Author(s) | Adarsh Naidu |
---|---|
Country | United States |
Abstract | Abstract This paper examines the utilization of Generative Adversarial Networks (GANs) for creating realistic synthetic financial market data to enhance the training of algorithmic trading models. Given that financial markets are highly dynamic and influenced by numerous factors, historical data often lacks sufficient coverage of rare occurrences and extreme volatility, limiting the predictive strength of trading models (Zhang, Aggarwal, & Qi, 2019). GANs, consisting of a generator and discriminator trained in an adversarial manner (Goodfellow et al., 2014), offer a potential solution by generating synthetic datasets that closely replicate actual market behaviors. This study presents a methodology where GANs are trained on historical price and volume data to create synthetic time series, subsequently improving model robustness and volatility prediction. Advantages include enhanced data variability, better forecasting accuracy, and the ability to simulate a range of market conditions. Quantitative analysis from case studies demonstrates a 15-20% enhancement in model performance metrics such as prediction accuracy and risk-adjusted returns (Hochreiter & Schmidhuber, 1997). Additionally, GANs facilitate stress testing and scenario analysis, which are essential for modern trading strategies. Future research should focus on refining GAN architectures for financial market specificity and addressing ethical concerns. This work highlights GANs' transformative potential in algorithmic trading by mitigating data limitations through advanced simulation. |
Field | Engineering |
Published In | Volume 2, Issue 5, September-October 2020 |
Published On | 2020-09-09 |
DOI | https://doi.org/10.36948/ijfmr.2020.v02i05.40783 |
Short DOI | https://doi.org/g9bvd4 |
Share this

E-ISSN 2582-2160

CrossRef DOI is assigned to each research paper published in our journal.
IJFMR DOI prefix is
10.36948/ijfmr
Downloads
All research papers published on this website are licensed under Creative Commons Attribution-ShareAlike 4.0 International License, and all rights belong to their respective authors/researchers.
