
International Journal For Multidisciplinary Research
E-ISSN: 2582-2160
•
Impact Factor: 9.24
A Widely Indexed Open Access Peer Reviewed Multidisciplinary Bi-monthly Scholarly International Journal
Home
Research Paper
Submit Research Paper
Publication Guidelines
Publication Charges
Upload Documents
Track Status / Pay Fees / Download Publication Certi.
Editors & Reviewers
View All
Join as a Reviewer
Get Membership Certificate
Current Issue
Publication Archive
Conference
Publishing Conf. with IJFMR
Upcoming Conference(s) ↓
WSMCDD-2025
GSMCDD-2025
Conferences Published ↓
ICCE (2025)
RBS:RH-COVID-19 (2023)
ICMRS'23
PIPRDA-2023
Contact Us
Plagiarism is checked by the leading plagiarism checker
Call for Paper
Volume 7 Issue 3
May-June 2025
Indexing Partners

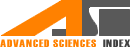
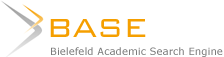



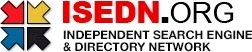

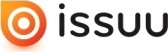

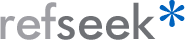
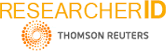
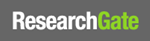
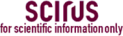
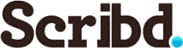


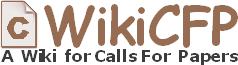

Adversarial Robustness of Credit Scoring Models - Leveraging GANs for Model Evaluation and Security
Author(s) | Adarsh Naidu |
---|---|
Country | United States |
Abstract | Credit scoring models play a crucial role in the financial industry by enabling lenders to assess creditworthiness efficiently. However, these machine learning-based models are susceptible to adversarial attacks, where fraudulent actors manipulate input data to trick the system into approving high-risk applicants. This study explores the application of Generative Adversarial Networks (GANs) in generating synthetic adversarial examples to evaluate the robustness of credit scoring models. By training GANs on historical credit data, we generate realistic adversarial samples that expose vulnerabilities in existing models. Experimental results demonstrate that these GAN-generated adversarial instances successfully mislead a standard credit scoring model in 25% of cases. To counteract this issue, we propose adversarial training as a defense mechanism, reducing the misclassification rate to 10%, thereby strengthening model resilience. This research highlights the importance of adversarial robustness in credit scoring models and introduces a novel framework for assessing and enhancing their security. The findings contribute to improving the reliability of credit decision-making while addressing regulatory compliance and fraud prevention challenges in the financial sector. Future research will focus on exploring advanced GAN architectures and establishing standardized benchmarks for robustness assessment. |
Field | Engineering |
Published In | Volume 1, Issue 1, July-August 2019 |
Published On | 2019-07-05 |
DOI | https://doi.org/10.36948/ijfmr.2019.v01i01.40956 |
Short DOI | https://doi.org/g9dgm9 |
Share this

E-ISSN 2582-2160

CrossRef DOI is assigned to each research paper published in our journal.
IJFMR DOI prefix is
10.36948/ijfmr
Downloads
All research papers published on this website are licensed under Creative Commons Attribution-ShareAlike 4.0 International License, and all rights belong to their respective authors/researchers.
