
International Journal For Multidisciplinary Research
E-ISSN: 2582-2160
•
Impact Factor: 9.24
A Widely Indexed Open Access Peer Reviewed Multidisciplinary Bi-monthly Scholarly International Journal
Home
Research Paper
Submit Research Paper
Publication Guidelines
Publication Charges
Upload Documents
Track Status / Pay Fees / Download Publication Certi.
Editors & Reviewers
View All
Join as a Reviewer
Get Membership Certificate
Current Issue
Publication Archive
Conference
Publishing Conf. with IJFMR
Upcoming Conference(s) ↓
WSMCDD-2025
GSMCDD-2025
Conferences Published ↓
RBS:RH-COVID-19 (2023)
ICMRS'23
PIPRDA-2023
Contact Us
Plagiarism is checked by the leading plagiarism checker
Call for Paper
Volume 7 Issue 2
March-April 2025
Indexing Partners

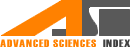
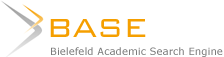



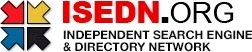

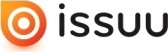

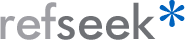
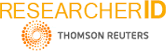
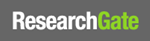
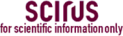
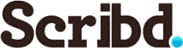


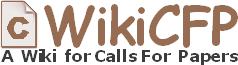

Federated Learning for Private Health Data Training
Author(s) | Vilas N |
---|---|
Country | India |
Abstract | The growing reliance on Artificial Intelligence (AI) in healthcare has brought about significant challenges related to privacy and security of patient data. Federated learning (FL) has emerged as a promising solution to these challenges by enabling collaborative model training without sharing sensitive data. This paper explores the application of federated learning in private health data training, outlining its principles, advantages, challenges, and future directions. Federated learning enables multiple institutions to collaborate in training machine learning models while keeping the data decentralized and protected. By maintaining data at local sites and sending only model updates to a central server, FL ensures that sensitive health information remains private and secure. The paper discusses how FL can be applied to a wide range of healthcare domains, including electronic health records (EHR), medical imaging, and predictive analytics. However, challenges such as data heterogeneity, communication inefficiencies, scalability issues, and the risk of adversarial attacks must be addressed. Additionally, ethical concerns related to patient consent, regulatory compliance, and data ownership are explored. The paper concludes by highlighting the potential of federated learning to revolutionize the way healthcare data is utilized for AI model training while preserving privacy, and it suggests ways to overcome the barriers for broader adoption in the healthcare sector. |
Field | Medical / Pharmacy |
Published In | Volume 7, Issue 2, March-April 2025 |
Published On | 2025-04-14 |
DOI | https://doi.org/10.36948/ijfmr.2025.v07i02.41434 |
Short DOI | https://doi.org/g9fm3j |
Share this

E-ISSN 2582-2160

CrossRef DOI is assigned to each research paper published in our journal.
IJFMR DOI prefix is
10.36948/ijfmr
Downloads
All research papers published on this website are licensed under Creative Commons Attribution-ShareAlike 4.0 International License, and all rights belong to their respective authors/researchers.
