
International Journal For Multidisciplinary Research
E-ISSN: 2582-2160
•
Impact Factor: 9.24
A Widely Indexed Open Access Peer Reviewed Multidisciplinary Bi-monthly Scholarly International Journal
Home
Research Paper
Submit Research Paper
Publication Guidelines
Publication Charges
Upload Documents
Track Status / Pay Fees / Download Publication Certi.
Editors & Reviewers
View All
Join as a Reviewer
Get Membership Certificate
Current Issue
Publication Archive
Conference
Publishing Conf. with IJFMR
Upcoming Conference(s) ↓
WSMCDD-2025
GSMCDD-2025
Conferences Published ↓
ICCE (2025)
RBS:RH-COVID-19 (2023)
ICMRS'23
PIPRDA-2023
Contact Us
Plagiarism is checked by the leading plagiarism checker
Call for Paper
Volume 7 Issue 3
May-June 2025
Indexing Partners

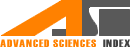
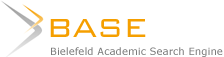



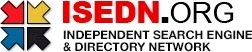

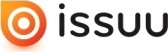

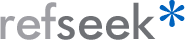
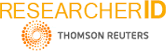
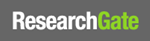
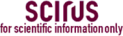
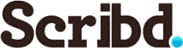


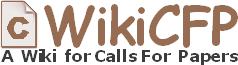

AI-Driven CPU Performance Profiling: Optimizing Power Efficiency and Computational Workloads
Author(s) | Mr. Manoj Chowdary Lingam, Mr. Ram Bhaskar Rakesh Marada |
---|---|
Country | United States |
Abstract | High performance computing in variety of industries has made the requirement of increasing CPU performance at the lowest power consumption more critical. The traditional profiling techniques, which are known to some extent, suffer in dynamically responding to the real time variations in the workload and the energy efficiency requirements. This paper discusses a paradigm shift in methods of improving power efficiency and managing computational workload using the AI-Driven CPU Performance Profiling. Machine learning algorithms and predictive analytics are integrated in AI based profiling and can be used to autonomously monitor, evaluate and optimize the processor performance parameters in real time. Various AI methodologies that are used for the detection of performance bottlenecks, prediction of thermal thresholds and balance of core utilization for varying into the study such as supervised learning, reinforcement learning, and deep neural networks. In addition, it studies how to extract lesson from previous performance data using AI models to produce actionable insights for task scheduling, thread allocation, and dynamic voltage and frequency scaling (DVFS) to decrease energy consumption overall while retaining the processing rate. In order to validate the effectiveness of AI driven profiling for various operational contexts, performance gains and energy savings are assessed in terms of key metrics (instructions per cycle (IPC), cache hit rates and power delay product). In addition to being an advancement in the field of intelligent hardware optimization, this research indicates that AI driven profiling not only leads to more efficient computation, but also integrates well with other sustainability goals of computing infrastructure. Finally, the paper recommends an integration of the AI profiling into next generation CPU architectures and development ecosystems. |
Keywords | CPU profiling, artificial intelligence, power efficiency, workload optimization, performance analytics |
Field | Computer > Artificial Intelligence / Simulation / Virtual Reality |
Published In | Volume 7, Issue 2, March-April 2025 |
Published On | 2025-04-24 |
DOI | https://doi.org/10.36948/ijfmr.2025.v07i02.41478 |
Short DOI | https://doi.org/g9gh8f |
Share this

E-ISSN 2582-2160

CrossRef DOI is assigned to each research paper published in our journal.
IJFMR DOI prefix is
10.36948/ijfmr
Downloads
All research papers published on this website are licensed under Creative Commons Attribution-ShareAlike 4.0 International License, and all rights belong to their respective authors/researchers.
