
International Journal For Multidisciplinary Research
E-ISSN: 2582-2160
•
Impact Factor: 9.24
A Widely Indexed Open Access Peer Reviewed Multidisciplinary Bi-monthly Scholarly International Journal
Home
Research Paper
Submit Research Paper
Publication Guidelines
Publication Charges
Upload Documents
Track Status / Pay Fees / Download Publication Certi.
Editors & Reviewers
View All
Join as a Reviewer
Get Membership Certificate
Current Issue
Publication Archive
Conference
Publishing Conf. with IJFMR
Upcoming Conference(s) ↓
WSMCDD-2025
GSMCDD-2025
AIMAR-2025
Conferences Published ↓
ICCE (2025)
RBS:RH-COVID-19 (2023)
ICMRS'23
PIPRDA-2023
Contact Us
Plagiarism is checked by the leading plagiarism checker
Call for Paper
Volume 7 Issue 4
July-August 2025
Indexing Partners

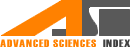
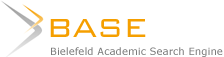



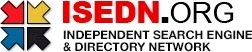

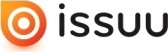

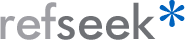
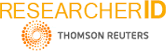
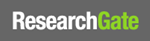
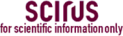
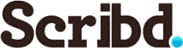


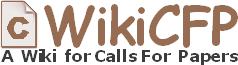

Enhancing Skin Disease Classification Through GAN-Generated Synthetic Images for Improved CNN Training and Generalization
Author(s) | Ms. Senjuti Ghosal, Dr.Senthil MuruganV, Neha Bharadwaj, Relli Naga Sai |
---|---|
Country | India |
Abstract | The research introduces GANs to CNNs for improving skin disease classification tasks. GANs produce synthetic realistic images of scarce skin lesions to tackle both data insufficiency and class imbalance problems. When using real and synthetic data for training the CNN model it generates better accuracy along with improved generalization mainly toward the identification of rare conditions including actinic keratosis and vascular lesions. The model performance receives enhancement from WeightedRandomSampler in addition to mixed precision training which achieves both class balancing and training acceleration. Advanced techniques and methods help the system produce accurate diagnoses and generalize to diagnose rare skin diseases more effectively. Data synthesis proves its worth as an addition to medical image classification operations by enabling fewer annotated medical image datasets. Flask and Streamlit enabled real-time deployment which creates an easy-to-use platform for healthcare staff allowing the solution to scale for professional use. The medical field can experience a breakthrough through this method which allows immediate and trustworthy identification of skin diseases. The solution provides meaningful benefits to populations that lack enough skilled dermatologists which results in enhanced healthcare results for patients. This project delivers an effective model which enables early accurate skin disease diagnosis that supports better patient care in dermatology fields |
Keywords | Skin Disease Classification, Generative Adversarial Networks (GANs), Convolutional Neural Networks (CNNs), Data Augmentation, Class Imbalance, Real-Time Inference, Mixed-Precision Training, WeightedRandomSampler, Synthetic Data, Early Diagnosis. |
Field | Engineering |
Published In | Volume 7, Issue 3, May-June 2025 |
Published On | 2025-05-11 |
DOI | https://doi.org/10.36948/ijfmr.2025.v07i03.41555 |
Short DOI | https://doi.org/g9kfgb |
Share this

E-ISSN 2582-2160

CrossRef DOI is assigned to each research paper published in our journal.
IJFMR DOI prefix is
10.36948/ijfmr
Downloads
All research papers published on this website are licensed under Creative Commons Attribution-ShareAlike 4.0 International License, and all rights belong to their respective authors/researchers.
