
International Journal For Multidisciplinary Research
E-ISSN: 2582-2160
•
Impact Factor: 9.24
A Widely Indexed Open Access Peer Reviewed Multidisciplinary Bi-monthly Scholarly International Journal
Home
Research Paper
Submit Research Paper
Publication Guidelines
Publication Charges
Upload Documents
Track Status / Pay Fees / Download Publication Certi.
Editors & Reviewers
View All
Join as a Reviewer
Get Membership Certificate
Current Issue
Publication Archive
Conference
Publishing Conf. with IJFMR
Upcoming Conference(s) ↓
WSMCDD-2025
GSMCDD-2025
Conferences Published ↓
ICCE (2025)
RBS:RH-COVID-19 (2023)
ICMRS'23
PIPRDA-2023
Contact Us
Plagiarism is checked by the leading plagiarism checker
Call for Paper
Volume 7 Issue 3
May-June 2025
Indexing Partners

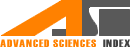
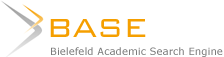



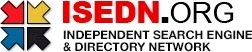

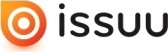

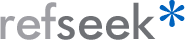
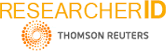
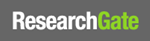
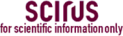
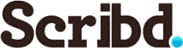


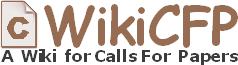

Deep Learning Models In Early Prediction And Risk Identification Of Chronic Kidney Disease
Author(s) | Mrs. Durggashre P, Ms. Narmatha D, Ms. Sabareeswari S, Ms. Swarna M |
---|---|
Country | India |
Abstract | Abstract— If not identified and treated promptly, chronic kidney disease (CKD), a gradual illness in which the kidneys lose their capacity to filter blood, can result in irreversible kidney failure. There has never been a greater need for effective diagnostic tools because prompt detection is essential to halting the disease's progression to more severe stages. Convolutional Neural Networks (CNNs), a type of deep learning technique, have demonstrated great potential in the early prediction and risk identification of chronic kidney disease (CKD) in recent years. These sophisticated models enable earlier detection than conventional techniques by automatically identifying intricate patterns and associations in enormous volumes of clinical data, such as patient demographics, test results, and medical imaging. This study investigates how deep learning models more especially, CNN scan be used to identify CKD in its early stages. We go over CNNs' fundamental design, their capacity to process intricate, multifaceted data, and how well they identify individuals who are at risk before the illness progresses to an irreparable point. Furthermore, we draw attention to the potential advantages of these models in enhancing patient outcomes by facilitating early intervention and treatment strategy optimization. The purpose of the work is to add to the expanding corpus of research on the application of AI in healthcare for the early detection and treatment of chronic illnesses such as chronic kidney disease. |
Keywords | Convolutional Neural Networks (CNN)), Deep Learning. |
Field | Engineering |
Published In | Volume 7, Issue 3, May-June 2025 |
Published On | 2025-05-10 |
Share this

E-ISSN 2582-2160

CrossRef DOI is assigned to each research paper published in our journal.
IJFMR DOI prefix is
10.36948/ijfmr
Downloads
All research papers published on this website are licensed under Creative Commons Attribution-ShareAlike 4.0 International License, and all rights belong to their respective authors/researchers.
