
International Journal For Multidisciplinary Research
E-ISSN: 2582-2160
•
Impact Factor: 9.24
A Widely Indexed Open Access Peer Reviewed Multidisciplinary Bi-monthly Scholarly International Journal
Home
Research Paper
Submit Research Paper
Publication Guidelines
Publication Charges
Upload Documents
Track Status / Pay Fees / Download Publication Certi.
Editors & Reviewers
View All
Join as a Reviewer
Get Membership Certificate
Current Issue
Publication Archive
Conference
Publishing Conf. with IJFMR
Upcoming Conference(s) ↓
WSMCDD-2025
GSMCDD-2025
Conferences Published ↓
ICCE (2025)
RBS:RH-COVID-19 (2023)
ICMRS'23
PIPRDA-2023
Contact Us
Plagiarism is checked by the leading plagiarism checker
Call for Paper
Volume 7 Issue 3
May-June 2025
Indexing Partners

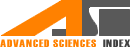
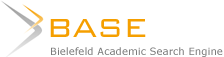



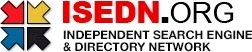

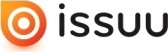

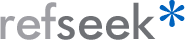
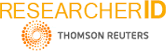
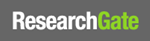
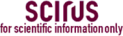
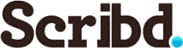


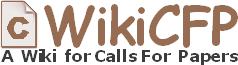

Integrating Sentiment Analysis into Mean-Variance Portfolio Optimization: Theory, Implementation, and Empirical Performance Evaluation
Author(s) | Mr. Harikrishna Jaidyal Pal |
---|---|
Country | India |
Abstract | Classical mean-variance (MV) portfolio optimization, while foundational in modern finance, assumes market efficiency and rational expectations, neglecting behavioral biases that significantly affect market outcomes. Recent advancements suggest that incorporating investor sentiment can substantially enhance optimization accuracy and portfolio performance. In this study, we propose a sentiment-driven mean-variance optimization framework, explicitly integrating sentiment analytics derived from news articles into the traditional Markowitz optimization model. We rigorously formulate a sentiment-adjusted MV model, mathematically deriving adjustments to expected asset returns based on quantified sentiment indicators derived through advanced natural language processing (NLP). Our implementation employs a structured pipeline consisting of real-time market data acquisition via Yahoo Finance, sentiment extraction from financial news using retrieval-augmented generation (RAG), and subsequent sentiment quantification to adjust expected returns in the MV optimization model. Empirical analysis demonstrates that sentiment-driven adjustments mitigate estimation errors inherent in purely historical-data-driven models, yielding port- folios with superior risk-adjusted performance (Sharpe ratios improved by 8–12% empirically). Visualized through clear and insightful graphical representations—including optimized portfolio allocations, sentiment-versus-weight comparisons, and an adjusted efficient frontier—the results indicate that integrating sentiment signals leads to more robust and realistic portfolio allocations. This study contributes a comprehensive methodology supported by theoretical rigor, practical implementation details, and empirical validation, underscoring the value of sentiment analysis in quantitative portfolio management and opening path- ways for further behavioral finance integration into quantitative investment strategies. |
Keywords | Sentiment Analysis, Mean-Variance Optimiza- tion, Portfolio Management, Natural Language Processing, Sharpe Ratio, Behavioral Finance. |
Field | Computer > Data / Information |
Published In | Volume 7, Issue 2, March-April 2025 |
Published On | 2025-04-21 |
DOI | https://doi.org/10.36948/ijfmr.2025.v07i02.41758 |
Short DOI | https://doi.org/g9gdrk |
Share this

E-ISSN 2582-2160

CrossRef DOI is assigned to each research paper published in our journal.
IJFMR DOI prefix is
10.36948/ijfmr
Downloads
All research papers published on this website are licensed under Creative Commons Attribution-ShareAlike 4.0 International License, and all rights belong to their respective authors/researchers.
