
International Journal For Multidisciplinary Research
E-ISSN: 2582-2160
•
Impact Factor: 9.24
A Widely Indexed Open Access Peer Reviewed Multidisciplinary Bi-monthly Scholarly International Journal
Home
Research Paper
Submit Research Paper
Publication Guidelines
Publication Charges
Upload Documents
Track Status / Pay Fees / Download Publication Certi.
Editors & Reviewers
View All
Join as a Reviewer
Get Membership Certificate
Current Issue
Publication Archive
Conference
Publishing Conf. with IJFMR
Upcoming Conference(s) ↓
WSMCDD-2025
GSMCDD-2025
Conferences Published ↓
RBS:RH-COVID-19 (2023)
ICMRS'23
PIPRDA-2023
Contact Us
Plagiarism is checked by the leading plagiarism checker
Call for Paper
Volume 7 Issue 2
March-April 2025
Indexing Partners

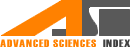
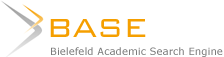



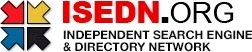

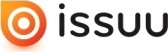

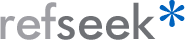
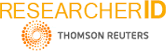
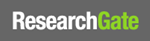
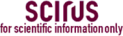
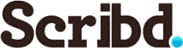


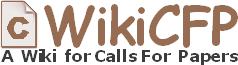

Regime Switching Models in Finance and Economics: Traditional Approaches And Machine Learning Enhancements
Author(s) | Pavan Mullapudi |
---|---|
Country | United States |
Abstract | Regime-switching models are a vital class of econometric and statistical tools that allow parameters or data-generating processes to change across different periods or “regimes.” This paper surveys traditional regime-switching approaches, including Markov-switching, threshold, and hidden Markov models, reviewing their applications in finance, investments, and economics. We summarize how these models capture phenomena like business cycles, market phases (bull/bear), volatility regimes, and interest rate dynamics. We highlight successful identification of regimes such as recessions versus expansions and distinct market return states. Furthermore, we explore the integration of machine learning (ML), particularly using feature importance from ensemble methods (e.g., random forests), to enhance regime identification and variable selection. We discuss the potential of these hybrid methods, citing recent research and case studies like early warning systems. The paper concludes with a comparative discussion of traditional versus ML-augmented approaches and outlines future directions for regime-switching analysis in the context of big data and complex financial systems. |
Keywords | Regime switching, Markov-switching models, threshold models, hidden Markov models, machine learning, feature importance, financial economics, business cycles, early warning systems. |
Field | Engineering |
Published In | Volume 7, Issue 2, March-April 2025 |
Published On | 2025-03-07 |
DOI | https://doi.org/10.36948/ijfmr.2025.v07i02.41894 |
Short DOI | https://doi.org/g9fmxt |
Share this

E-ISSN 2582-2160

CrossRef DOI is assigned to each research paper published in our journal.
IJFMR DOI prefix is
10.36948/ijfmr
Downloads
All research papers published on this website are licensed under Creative Commons Attribution-ShareAlike 4.0 International License, and all rights belong to their respective authors/researchers.
