
International Journal For Multidisciplinary Research
E-ISSN: 2582-2160
•
Impact Factor: 9.24
A Widely Indexed Open Access Peer Reviewed Multidisciplinary Bi-monthly Scholarly International Journal
Home
Research Paper
Submit Research Paper
Publication Guidelines
Publication Charges
Upload Documents
Track Status / Pay Fees / Download Publication Certi.
Editors & Reviewers
View All
Join as a Reviewer
Get Membership Certificate
Current Issue
Publication Archive
Conference
Publishing Conf. with IJFMR
Upcoming Conference(s) ↓
WSMCDD-2025
GSMCDD-2025
AIMAR-2025
Conferences Published ↓
ICCE (2025)
RBS:RH-COVID-19 (2023)
ICMRS'23
PIPRDA-2023
Contact Us
Plagiarism is checked by the leading plagiarism checker
Call for Paper
Volume 7 Issue 4
July-August 2025
Indexing Partners

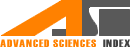
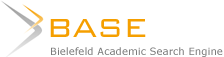



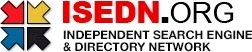

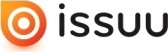

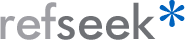
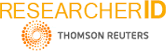
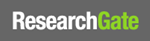
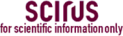
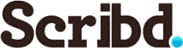


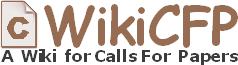

“Adaptive Control of Smart Hydroponic Systems Using Reinforcement Learning: A Comparative Study with PID”
Author(s) | Dr. Selvamani Dakshinamurthy, Dr. Baskar Mohan |
---|---|
Country | India |
Abstract | Abstract: Accuracy control in hydroponics systems is indispensable for achieving higher crop yield with lower resource utilization. Conventional control strategies like Proportional-Integral-Derivative (PID).controllers, while steadfast, may not possess the requisite adaptability to best suit intricate and dynamic horticultural environments. This work presents a reinforcement learning (RL)-driven control scheme as a smart replacement for PID in controlling major parameters in the GymHydro hydroponic system, namely lighting, watering, and fertilizer supply The RL setting was simulated using continuous action and state spaces, including environmental and plant growth parameters. More sophisticated algorithms like Proximal Policy Optimization (PPO) and Deep Deterministic Policy Gradient (DDPG) were tested in simulation, with reward functions optimized to support plant health, productivity, and system efficiency. During a six-month simulation duration, the RL-regulated system outperformed the PID-regulated baseline with 12.5% more crop yield, a 10–12.5% decrease in water, energy, and nutrient consumption, and a 3% increase in system uptime. All of these gains were significantly different (p ≤ 0.05). These findings demonstrate the capability of reinforcement learning to facilitate adaptive, data-driven optimization in smart agriculture. The suggested method not only enhances productivity but also aids sustainability objectives by minimizing operational costs and resource consumption. Real-world deployment and integration with IoT platforms will be investigated in future work to further improve robustness and scalability. |
Keywords | Keywords: Hydroponics, Reinforcement Learning, PID Control, Smart Agriculture, GymHydro, Energy Efficiency, Precision Farming |
Field | Computer Applications |
Published In | Volume 7, Issue 3, May-June 2025 |
Published On | 2025-05-08 |
DOI | https://doi.org/10.36948/ijfmr.2025.v07i03.42424 |
Short DOI | https://doi.org/g9hsk3 |
Share this

E-ISSN 2582-2160

CrossRef DOI is assigned to each research paper published in our journal.
IJFMR DOI prefix is
10.36948/ijfmr
Downloads
All research papers published on this website are licensed under Creative Commons Attribution-ShareAlike 4.0 International License, and all rights belong to their respective authors/researchers.
