
International Journal For Multidisciplinary Research
E-ISSN: 2582-2160
•
Impact Factor: 9.24
A Widely Indexed Open Access Peer Reviewed Multidisciplinary Bi-monthly Scholarly International Journal
Home
Research Paper
Submit Research Paper
Publication Guidelines
Publication Charges
Upload Documents
Track Status / Pay Fees / Download Publication Certi.
Editors & Reviewers
View All
Join as a Reviewer
Get Membership Certificate
Current Issue
Publication Archive
Conference
Publishing Conf. with IJFMR
Upcoming Conference(s) ↓
WSMCDD-2025
GSMCDD-2025
AIMAR-2025
Conferences Published ↓
ICCE (2025)
RBS:RH-COVID-19 (2023)
ICMRS'23
PIPRDA-2023
Contact Us
Plagiarism is checked by the leading plagiarism checker
Call for Paper
Volume 7 Issue 4
July-August 2025
Indexing Partners

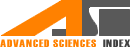
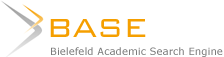



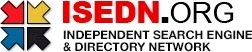

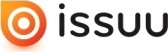

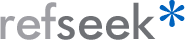
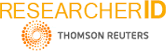
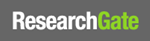
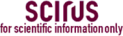
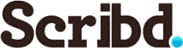


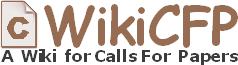

A Review on Hybrid Feature Selection Based Deep Learning Model for Enhanced Email Spam Detection
Author(s) | Ms. Neelam Banjare, Dr. Pranjali Gani |
---|---|
Country | India |
Abstract | Email spam detection remains a significant challenge in the field of cyber security and digital communication. As spam tactics grow increasingly sophisticated, there is a pressing need for more efficient, adaptable, and accurate spam detection systems. This research addresses these needs by proposing a novel hybrid approach that combines advanced feature selection techniques with deep learning for effective spam classification. Specifically, the study introduces a hybrid feature selection method that integrates correlation-based filtering with a genetic algorithm (GA) for optimizing the feature set, and deep learning models for classification. The primary goal of this research is to improve the accuracy and efficiency of spam detection systems by handling the challenges of high-dimensional data, redundant features, and the evolving nature of spam content. The feature selection process removes irrelevant and redundant features, ensuring that only the most important ones are used, thus reducing the complexity and computational cost of the model. Once the feature set is optimized, deep learning model is employed to classify emails as spam or non-spam, leveraging its ability to learn complex patterns from the data. The proposed method is evaluated on the Enron Email Spam Dataset, where it demonstrates superior performance compared to traditional machine learning models like Naïve Bayes, Support Vector Machines (SVM), and Random Forest, as well as other deep learning methods without feature selection. The results show that the hybrid approach achieves an accuracy of 97.82%, with a precision of 96.91%, recall of 98.26%, and an F1-score of 97.58%. Additionally, the Area under the Receiver Operating Characteristic Curve (AUC) reaches 0.987, indicating excellent performance in distinguishing spam from legitimate emails. This research highlights the effectiveness of combining robust feature selection techniques with deep learning models for spam classification. The results suggest that this hybrid approach is not only accurate but also efficient, capable of handling high-dimensional, noisy datasets. Moving forward, future research will focus on expanding the model's capabilities, such as incorporating online learning, handling multimodal spam (e.g., images and attachments), and adapting the system to work across multiple languages. Moreover, incorporating explainable AI (XAI) techniques will improve the transparency of the classification process, making the model more interpretable. In conclusion, this work offers valuable insights into the power of hybrid models in spam detection and sets the foundation for the development of more adaptive, scalable, and accurate systems to address the growing challenge of email spam. |
Keywords | Email Spam Detection, Feature Selection, Hybrid Method, Deep Learning, Spam Classification. |
Field | Engineering |
Published In | Volume 7, Issue 2, March-April 2025 |
Published On | 2025-04-26 |
DOI | https://doi.org/10.36948/ijfmr.2025.v07i02.42713 |
Short DOI | https://doi.org/g9gvgm |
Share this

E-ISSN 2582-2160

CrossRef DOI is assigned to each research paper published in our journal.
IJFMR DOI prefix is
10.36948/ijfmr
Downloads
All research papers published on this website are licensed under Creative Commons Attribution-ShareAlike 4.0 International License, and all rights belong to their respective authors/researchers.
