
International Journal For Multidisciplinary Research
E-ISSN: 2582-2160
•
Impact Factor: 9.24
A Widely Indexed Open Access Peer Reviewed Multidisciplinary Bi-monthly Scholarly International Journal
Home
Research Paper
Submit Research Paper
Publication Guidelines
Publication Charges
Upload Documents
Track Status / Pay Fees / Download Publication Certi.
Editors & Reviewers
View All
Join as a Reviewer
Get Membership Certificate
Current Issue
Publication Archive
Conference
Publishing Conf. with IJFMR
Upcoming Conference(s) ↓
WSMCDD-2025
GSMCDD-2025
Conferences Published ↓
RBS:RH-COVID-19 (2023)
ICMRS'23
PIPRDA-2023
Contact Us
Plagiarism is checked by the leading plagiarism checker
Call for Paper
Volume 7 Issue 2
March-April 2025
Indexing Partners

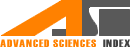
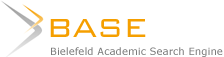



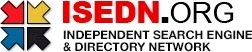

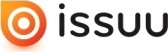

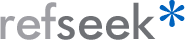
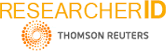
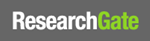
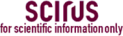
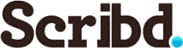


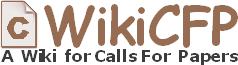

AI-Enabled Predictive Analytics in US Healthcare Leveraging Cloud-Based Data Warehousing for Disease Forecasting
Author(s) | Praveen Kumar Rawat |
---|---|
Country | United States |
Abstract | Integrating prediction analytics via AI and cloud-based data warehouse will transform disease prediction in the U.S. healthcare system with real-time, data-driven decision-making. This paper proposes a Hybrid Deep Learning Model that combines Long Short-Term Memory (LSTM) networks with Temporal Fusion Transform (TFT) and XGBoost to improve forecasting disease trends, early diagnosis of disease, and optimizing the health care resource allocation. It collects relevant insights while keeping their privacy safeguarded through federated learning from heterogeneous data sources, such as electronic health records (EHRs), wearable sensors data, and epidemiological reports. The proposed architecture of cloud integrated AI increases dynamic scalability and computational performance, harnessing Auto-ML for hyperparameter optimization and edge computing approach for real-time inference. Cross-validation on the real health care datasets confirmed that the proposed model is better in terms of performance on forecasting events, prediction latency, and resource utilization. Performance metrics showed that the TFT model attention mechanism enhances the feature selectivity, thus ensuring accuracy trend forecasting of chronic diseases such as diabetes, cardiovascular diseases, and respiratory diseases. The findings suggest that Hybrid Deep Learning Model outshines conventional machine learning techniques in predictive validity and explainability. AI-driven and cloud-hosted predictive analytics research shows promise in transforming public health intervention and reducing hospitalizations in patient outcomes. |
Field | Engineering |
Published In | Volume 7, Issue 1, January-February 2025 |
Published On | 2025-02-05 |
DOI | https://doi.org/10.36948/ijfmr.2025.v07i01.42902 |
Short DOI | https://doi.org/g9gdqf |
Share this

E-ISSN 2582-2160

CrossRef DOI is assigned to each research paper published in our journal.
IJFMR DOI prefix is
10.36948/ijfmr
Downloads
All research papers published on this website are licensed under Creative Commons Attribution-ShareAlike 4.0 International License, and all rights belong to their respective authors/researchers.
