
International Journal For Multidisciplinary Research
E-ISSN: 2582-2160
•
Impact Factor: 9.24
A Widely Indexed Open Access Peer Reviewed Multidisciplinary Bi-monthly Scholarly International Journal
Home
Research Paper
Submit Research Paper
Publication Guidelines
Publication Charges
Upload Documents
Track Status / Pay Fees / Download Publication Certi.
Editors & Reviewers
View All
Join as a Reviewer
Get Membership Certificate
Current Issue
Publication Archive
Conference
Publishing Conf. with IJFMR
Upcoming Conference(s) ↓
WSMCDD-2025
GSMCDD-2025
AIMAR-2025
Conferences Published ↓
ICCE (2025)
RBS:RH-COVID-19 (2023)
ICMRS'23
PIPRDA-2023
Contact Us
Plagiarism is checked by the leading plagiarism checker
Call for Paper
Volume 7 Issue 4
July-August 2025
Indexing Partners

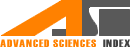
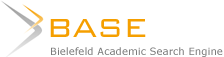



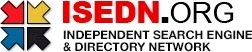

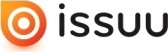

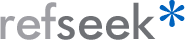
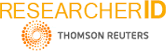
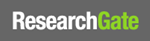
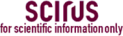
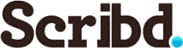


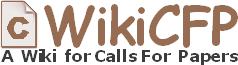

Adversarial Machine Learning Defenses in AI-Enabled Cybersecurity Systems
Author(s) | Mr. Chandrashekhar Moharir, Mr. Shivaraj Yanamandram Kuppuraju, Mr. Sambhav Patil |
---|---|
Country | India |
Abstract | This paper explores the effectiveness of adversarial machine learning (AML) defense strategies in enhancing the resilience of AI-enabled cybersecurity systems against sophisticated adversarial attacks. With the rapid adoption of AI in security-critical domains, ensuring model robustness has become paramount, particularly in the face of threats such as gradient-based and query-based adversarial perturbations. The study evaluates five widely recognized defense mechanisms—adversarial training, defensive distillation, gradient masking, ensemble learning, and input preprocessing—across key performance metrics including accuracy, precision, recall, F1-score, and robustness. Experimental results demonstrate that while each defense offers varying degrees of protection, ensemble learning consistently outperforms others, achieving the highest robustness and detection performance. The findings reveal that no single method can provide complete immunity, but strategic combinations and layered defenses offer substantial improvements in adversarial resistance. This research contributes to the understanding of AML defenses, guiding the development of more secure and dependable AI-driven cybersecurity systems. |
Keywords | Adversarial, Machine Learning, Defenses, Cybersecurity Systems, Deep Learning, Cyberattacks |
Field | Computer > Artificial Intelligence / Simulation / Virtual Reality |
Published In | Volume 7, Issue 2, March-April 2025 |
Published On | 2025-04-27 |
DOI | https://doi.org/10.36948/ijfmr.2025.v07i02.43075 |
Short DOI | https://doi.org/g9gvmt |
Share this

E-ISSN 2582-2160

CrossRef DOI is assigned to each research paper published in our journal.
IJFMR DOI prefix is
10.36948/ijfmr
Downloads
All research papers published on this website are licensed under Creative Commons Attribution-ShareAlike 4.0 International License, and all rights belong to their respective authors/researchers.
