
International Journal For Multidisciplinary Research
E-ISSN: 2582-2160
•
Impact Factor: 9.24
A Widely Indexed Open Access Peer Reviewed Multidisciplinary Bi-monthly Scholarly International Journal
Home
Research Paper
Submit Research Paper
Publication Guidelines
Publication Charges
Upload Documents
Track Status / Pay Fees / Download Publication Certi.
Editors & Reviewers
View All
Join as a Reviewer
Get Membership Certificate
Current Issue
Publication Archive
Conference
Publishing Conf. with IJFMR
Upcoming Conference(s) ↓
WSMCDD-2025
GSMCDD-2025
AIMAR-2025
Conferences Published ↓
ICCE (2025)
RBS:RH-COVID-19 (2023)
ICMRS'23
PIPRDA-2023
Contact Us
Plagiarism is checked by the leading plagiarism checker
Call for Paper
Volume 7 Issue 4
July-August 2025
Indexing Partners

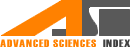
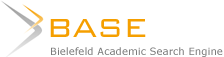



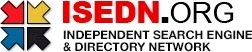

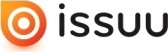

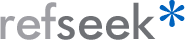
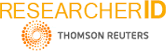
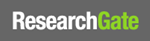
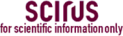
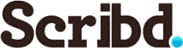


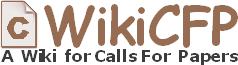

Deep Learning-Based Data Leak Prevention Systems for Enterprise Environments
Author(s) | Mr. Greesham Anand, Mr. Shivaraj Yanamandram Kuppuraju, Mr. Sambhav Patil |
---|---|
Country | India |
Abstract | This paper presents a novel approach to enhancing Data Leak Prevention (DLP) systems within enterprise environments through the application of deep learning techniques. Traditional DLP methods often struggle to effectively detect complex data exfiltration patterns and adapt to evolving threats, particularly when dealing with unstructured data formats and insider risks. To address these challenges, the proposed system leverages advanced deep learning models, including Convolutional Neural Networks (CNNs) and Bidirectional Long Short-Term Memory (BiLSTM) networks, to analyze structured and unstructured enterprise data in real-time. The system demonstrates high performance across key evaluation metrics, achieving an accuracy of 94.7%, precision of 93.1%, recall of 91.5%, F1-score of 92.3%, and AUC-ROC of 96.1%, significantly outperforming traditional DLP approaches. The integration of natural language processing and behavioral analytics enhances the system’s ability to detect sensitive data leaks with greater context awareness and minimal false positives. Additionally, the methodology incorporates real-world enterprise datasets and adheres to regulatory compliance standards, ensuring practical applicability and legal alignment. The findings underscore the potential of deep learning to transform enterprise data protection strategies by providing scalable, adaptive, and intelligent DLP solutions. |
Keywords | Deep Learning, Data Leak Prevention, Enterprise Security, Natural Language Processing, Insider Threat Detection. |
Field | Engineering |
Published In | Volume 7, Issue 2, March-April 2025 |
Published On | 2025-04-27 |
DOI | https://doi.org/10.36948/ijfmr.2025.v07i02.43098 |
Short DOI | https://doi.org/g9gvmn |
Share this

E-ISSN 2582-2160

CrossRef DOI is assigned to each research paper published in our journal.
IJFMR DOI prefix is
10.36948/ijfmr
Downloads
All research papers published on this website are licensed under Creative Commons Attribution-ShareAlike 4.0 International License, and all rights belong to their respective authors/researchers.
