
International Journal For Multidisciplinary Research
E-ISSN: 2582-2160
•
Impact Factor: 9.24
A Widely Indexed Open Access Peer Reviewed Multidisciplinary Bi-monthly Scholarly International Journal
Home
Research Paper
Submit Research Paper
Publication Guidelines
Publication Charges
Upload Documents
Track Status / Pay Fees / Download Publication Certi.
Editors & Reviewers
View All
Join as a Reviewer
Get Membership Certificate
Current Issue
Publication Archive
Conference
Publishing Conf. with IJFMR
Upcoming Conference(s) ↓
WSMCDD-2025
GSMCDD-2025
AIMAR-2025
Conferences Published ↓
ICCE (2025)
RBS:RH-COVID-19 (2023)
ICMRS'23
PIPRDA-2023
Contact Us
Plagiarism is checked by the leading plagiarism checker
Call for Paper
Volume 7 Issue 4
July-August 2025
Indexing Partners

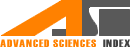
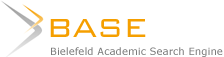



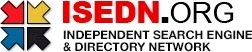

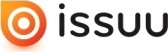

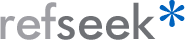
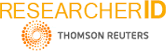
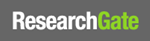
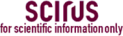
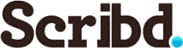


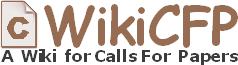

Object Detection in Low-Light Conditions Using YOLOV7
Author(s) | Ms. Hujaimah Nida |
---|---|
Country | India |
Abstract | Reduced contrast, increased noise, and shifting lighting levels are some of the factors that make object recognition in low light circumstances extremely difficult. Accurate identification in these situations is essential for applications where reliable performance is essential, such as driverless cars, security monitoring, and surveillance systems. This work makes use of the cutting-edge YOLOv7 object recognition model, It is renowned for its remarkable real-time processing speed and precision. The model is trained and evaluated using the ExDark dataset, which comprises twelve different object categories captured in different low-light scenarios.Adaptive histogram equalization and picture normalization are two preprocessing techniques used to enhance image quality and boost detection efficiency before to feeding the data into the YOLOv7 model. Training is done using pre-trained weights from the COCO dataset, which has been specially adapted to recognize objects in low light. The model is evaluated using standard assessment criteria, such as mean average precision (mAP), recall, and precision. With a recall of 0.6556 and a precision of 0.7153, YOLOv7 performs better on the same dataset than previous iterations such as YOLOv5 and IA-YOLO, according to the experimental results. The advanced network architecture of YOLOv7, which incorporates trainable Bag-of-Freebies and E-ELAN modules to enable efficient Feature extraction in low light, is credited with the enhanced performance. The results highlight YOLOv7's potential for useful implementation in applications that need precise object detection in difficult illumination conditions. To further maximize real-time performance on edge devices, future improvements might include incorporating sophisticated picture augmentation techniques, image enhancement algorithms, and lightweight designs. The importance of continuously improving object detection technology is highlighted in this study in order to overcome the ongoing problems with low-light detection. |
Keywords | YOLOV7, deep learning, object detection, low-light video, ExDark dataset, Autonomous surveillance, Mean Average Precision (mAP). |
Field | Computer Applications |
Published In | Volume 7, Issue 3, May-June 2025 |
Published On | 2025-05-04 |
DOI | https://doi.org/10.36948/ijfmr.2025.v07i03.43710 |
Short DOI | https://doi.org/g9hsht |
Share this

E-ISSN 2582-2160

CrossRef DOI is assigned to each research paper published in our journal.
IJFMR DOI prefix is
10.36948/ijfmr
Downloads
All research papers published on this website are licensed under Creative Commons Attribution-ShareAlike 4.0 International License, and all rights belong to their respective authors/researchers.
