
International Journal For Multidisciplinary Research
E-ISSN: 2582-2160
•
Impact Factor: 9.24
A Widely Indexed Open Access Peer Reviewed Multidisciplinary Bi-monthly Scholarly International Journal
Home
Research Paper
Submit Research Paper
Publication Guidelines
Publication Charges
Upload Documents
Track Status / Pay Fees / Download Publication Certi.
Editors & Reviewers
View All
Join as a Reviewer
Get Membership Certificate
Current Issue
Publication Archive
Conference
Publishing Conf. with IJFMR
Upcoming Conference(s) ↓
WSMCDD-2025
GSMCDD-2025
AIMAR-2025
Conferences Published ↓
ICCE (2025)
RBS:RH-COVID-19 (2023)
ICMRS'23
PIPRDA-2023
Contact Us
Plagiarism is checked by the leading plagiarism checker
Call for Paper
Volume 7 Issue 4
July-August 2025
Indexing Partners

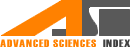
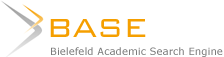



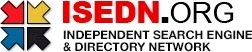

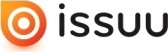

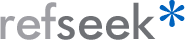
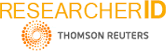
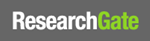
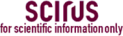
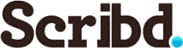


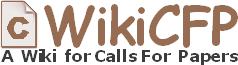

Botnet Detection in IOT Using Unsupervised Learning Techniques DBSCAN and ANN
Author(s) | Mr. Naresh kumar K, Dr. Prathapchandran K |
---|---|
Country | India |
Abstract | The rapid proliferation of Internet of Things (IoT) devices has significantly increased the attack surface for cyber threats, notably botnets. Traditional detection methods often fall short in identifying sophisticated and evolving botnet behaviors. This research explores the efficacy of unsupervised learning techniques, specifically Density-Based Spatial Clustering of Applications with Noise (DBSCAN) and Artificial Neural Networks (ANN), for botnet detection in IoT environments. DBSCAN is leveraged for its ability to identify anomalies in network traffic data without predefined labels, while ANN is utilized for its capacity to recognize complex patterns and adapt to new threats. The proposed framework combines the strengths of DBSCAN in clustering and noise handling with the adaptive learning capabilities of ANN. This hybrid approach is designed to detect both known and unknown botnet activities, providing a more dynamic and responsive security solution. Extensive experiments were conducted on diverse IoT datasets, encompassing various types of network traffic and botnet behaviors. The results indicate that the integrated DBSCAN-ANN model outperforms traditional detection methods in terms of accuracy, precision, and recall, while maintaining low false-positive rates. Furthermore, the study delves into the computational efficiency of the proposed model, demonstrating its scalability and suitability for real-time deployment in resource-constrained IoT environments. The results emphasize the potential of unsupervised learning techniques to enhance IoT security by offering a proactive and adaptive defense mechanism against the ever-evolving threat landscape posed by botnets. This research contributes to the ongoing efforts in securing IoT ecosystems and underscores the importance of innovative machine learning applications in cybersecurity. |
Keywords | Botnet, IoT traffic, cyber attack, benign, malicious, machine learning, deep learning, accuracy, precision, recall and F1-Score. |
Field | Computer Applications |
Published In | Volume 7, Issue 3, May-June 2025 |
Published On | 2025-05-08 |
DOI | https://doi.org/10.36948/ijfmr.2025.v07i03.43726 |
Short DOI | https://doi.org/g9hsm7 |
Share this

E-ISSN 2582-2160

CrossRef DOI is assigned to each research paper published in our journal.
IJFMR DOI prefix is
10.36948/ijfmr
Downloads
All research papers published on this website are licensed under Creative Commons Attribution-ShareAlike 4.0 International License, and all rights belong to their respective authors/researchers.
