
International Journal For Multidisciplinary Research
E-ISSN: 2582-2160
•
Impact Factor: 9.24
A Widely Indexed Open Access Peer Reviewed Multidisciplinary Bi-monthly Scholarly International Journal
Home
Research Paper
Submit Research Paper
Publication Guidelines
Publication Charges
Upload Documents
Track Status / Pay Fees / Download Publication Certi.
Editors & Reviewers
View All
Join as a Reviewer
Get Membership Certificate
Current Issue
Publication Archive
Conference
Publishing Conf. with IJFMR
Upcoming Conference(s) ↓
WSMCDD-2025
GSMCDD-2025
Conferences Published ↓
ICCE (2025)
RBS:RH-COVID-19 (2023)
ICMRS'23
PIPRDA-2023
Contact Us
Plagiarism is checked by the leading plagiarism checker
Call for Paper
Volume 7 Issue 3
May-June 2025
Indexing Partners

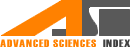
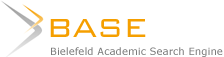



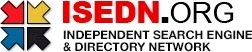

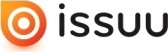

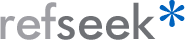
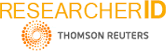
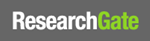
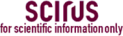
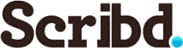


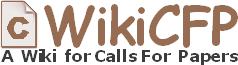

Machine Learning-Based Cybersecurity in Advanced Autonomous and Connected Vehicles
Author(s) | Ms. Tejal Ramesh Borkar, Prof. Dr. Nikita Hatwar, Mr. Prashik Vilas Lamsoge, Mr. Vedant Umesh Kapgate, Mr. Anurag Anil Yamnurwar, Mr. Ashay Pramod Wanjari |
---|---|
Country | India |
Abstract | Abstract—The rapid growth of Advanced Autonomous and Connected Vehicles (AACVs) has been revolutionizing transport while building significant cybersecurity risks. AACVs relies on Electronic Control Units (ECUs), networked sensors, vehicle-to-everything (V2X) networks, and cloud facilities, which makes them vulnerable to replay attacks, GPS spoofing, Man-in-the-Middle (MITM) attacks, malware injection, and denial-of-service (DoS) attacks. Smart key systems have been compromised using software-defined radio (SDR) tools such as HackRF One to offer unauthorized access through RF signal replay. Similarly, laser interference with LiDAR and camera sensors has shown the capability to disrupt autonomous navigation. To address such threats, machine learning (ML)-based techniques are employed for anomaly detection, predictive threat analysis, and intrusion prevention. The proposed framework uses decision trees, ensemble models, and generative adversarial networks (GANs) in combination to detect cyberattacks in real time. Federated learning is utilized to preserve data privacy through facilitating joint model training on various vehicles without sharing sensitive raw data with central servers.An intelligent multi-stage intrusion detection system (IDS) is utilized, combining rule-based filtering and ML classifiers for low-latency, high-accuracy threat detection. Experimental evidence indicates that the use of ML for anomaly detection reduces the impacts of replay attacks, network intrusion, and spoofing of sensors. Other safeguards like secure diagnostic protocols, biometric authentication, and encryption are incorporated to deter zero-day attacks in AACVs. |
Keywords | Keywords—Autonomous Vehicles, Intrusion Detection, Software Defined Radio, Generative Adversarial Networks, Machine Learning, GPS Spoofing, Cybersecurity, CAN Bus, Smart Key Attacks, LiDAR Disruption |
Field | Engineering |
Published In | Volume 7, Issue 3, May-June 2025 |
Published On | 2025-05-08 |
DOI | https://doi.org/10.36948/ijfmr.2025.v07i03.43962 |
Short DOI | https://doi.org/g9hsjw |
Share this

E-ISSN 2582-2160

CrossRef DOI is assigned to each research paper published in our journal.
IJFMR DOI prefix is
10.36948/ijfmr
Downloads
All research papers published on this website are licensed under Creative Commons Attribution-ShareAlike 4.0 International License, and all rights belong to their respective authors/researchers.
