
International Journal For Multidisciplinary Research
E-ISSN: 2582-2160
•
Impact Factor: 9.24
A Widely Indexed Open Access Peer Reviewed Multidisciplinary Bi-monthly Scholarly International Journal
Home
Research Paper
Submit Research Paper
Publication Guidelines
Publication Charges
Upload Documents
Track Status / Pay Fees / Download Publication Certi.
Editors & Reviewers
View All
Join as a Reviewer
Get Membership Certificate
Current Issue
Publication Archive
Conference
Publishing Conf. with IJFMR
Upcoming Conference(s) ↓
WSMCDD-2025
GSMCDD-2025
AIMAR-2025
Conferences Published ↓
ICCE (2025)
RBS:RH-COVID-19 (2023)
ICMRS'23
PIPRDA-2023
Contact Us
Plagiarism is checked by the leading plagiarism checker
Call for Paper
Volume 7 Issue 4
July-August 2025
Indexing Partners

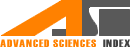
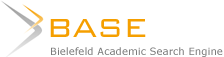



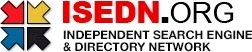

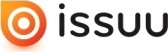

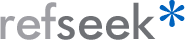
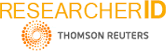
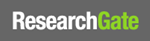
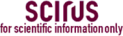
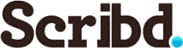


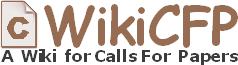

Semantic Segmentation of Ocular Regions Using Artificial Intelligence for Biometric and Medical Uses
Author(s) | Dr. Nirgish Kumar, Komal, Dr. C.S. Raghuvamshi |
---|---|
Country | India |
Abstract | Segmenting multiple ocular regions is crucial for a variety of applications, including gaze estimation, liveness detection, biometrics, and healthcare. Segmentation methods usually concentrate on one area of the eye at a time. Very little research has been done on some areas of the eye, despite the many clear benefits. Similarly, in difficult situations with blur, ghost effects, low resolution, off-angles, and odd glints, precise segmentation of several eye regions is required. These limitations cannot currently be addressed by the segmentation techniques that are available. This research proposes a lightweight outer residual encoder-decoder network that can be used with a variety of sensor images in order to accurately segment multiple eye regions in unconstrained circumstances. The suggested technique uses the high-frequency information flow from the outer residual encoder-decoder deep convolutional neural network (named ORED-Net) to identify the actual borders of the eye regions from low-quality photos. Furthermore, the performance of the suggested ORED-Net model is not enhanced by network depth, complexity, or parameter count. The suggested network weighs significantly less than earlier cutting-edge models. Extensive studies were conducted utilizing the SBVPI and UBIRIS.v2 datasets, which contain images of the eye Semantic Segmentation of Ocular Regions Using Artificial Intelligence for Biometric and Medical Uses The Tech Science Pressgion, and ideal performance was attained. The mean intersection over union score (mIoU) of the simulation results produced by the suggested ORED Net on the difficult SBVPI and UBIRIS.v2 datasets was 89.77 and 87.27, respectively. |
Keywords | Keywords: Deep Learning, Sensors, Biometrics, Medical Uses, Semantic Segmentation, and Ocular Regions. |
Field | Computer > Artificial Intelligence / Simulation / Virtual Reality |
Published In | Volume 7, Issue 3, May-June 2025 |
Published On | 2025-05-13 |
DOI | https://doi.org/10.36948/ijfmr.2025.v07i03.44282 |
Short DOI | https://doi.org/g9kfkx |
Share this

E-ISSN 2582-2160

CrossRef DOI is assigned to each research paper published in our journal.
IJFMR DOI prefix is
10.36948/ijfmr
Downloads
All research papers published on this website are licensed under Creative Commons Attribution-ShareAlike 4.0 International License, and all rights belong to their respective authors/researchers.
