
International Journal For Multidisciplinary Research
E-ISSN: 2582-2160
•
Impact Factor: 9.24
A Widely Indexed Open Access Peer Reviewed Multidisciplinary Bi-monthly Scholarly International Journal
Home
Research Paper
Submit Research Paper
Publication Guidelines
Publication Charges
Upload Documents
Track Status / Pay Fees / Download Publication Certi.
Editors & Reviewers
View All
Join as a Reviewer
Get Membership Certificate
Current Issue
Publication Archive
Conference
Publishing Conf. with IJFMR
Upcoming Conference(s) ↓
WSMCDD-2025
GSMCDD-2025
AIMAR-2025
Conferences Published ↓
ICCE (2025)
RBS:RH-COVID-19 (2023)
ICMRS'23
PIPRDA-2023
Contact Us
Plagiarism is checked by the leading plagiarism checker
Call for Paper
Volume 7 Issue 4
July-August 2025
Indexing Partners

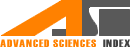
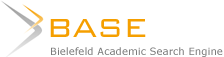



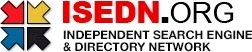

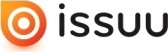

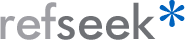
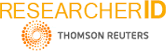
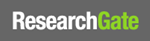
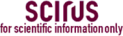
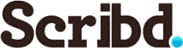


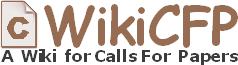

Optimizing Text Summarization and Content Tagging: A Performance Comparison of General Purpose Large Language Models and Specialized Architectures
Author(s) | Mr. Bosco Chanam, Mr. Ashay Kumar Singh, Mr. Chris Dcosta, Mr. Arghadeep Das, Prof. Dr. Shwetambari Chiwhane |
---|---|
Country | India |
Abstract | In NLP, Text summarization and content tagging are essential problems that are dedicated to improving information accessibility and organization. Summarization reduces the quantity of information that has to be stored or transmitted, and content tagging enables information to be stored in categories.This project focuses on enhancing two critical tasks in natural language processing: Text summarization and content tagging are the two most typical applications of text comprehension. In the first task, the text summarization is accomplished with the help of a general-purpose large language model (LLM). It is then compared with other similar models for the purpose of summarization to check out for any enhancements in accuracy, coherence and relevance. This is in an effort to understand the efficiency of fine-tuning a general LLM compared to the application of task-specific models to fine-tune and improve text summarization for various usages. In the second task, content tagging, the BERT model is used on a classification data set where it is working on the specific task of labeling the given content with the appropriate tags. Then, the performance of the proposed BERT is compared with other classification models that were also presented in the research group and discussed earlier. The purpose here is to examine how effectively models can work in terms of being accurate, fast and smart in identifying content in line with context and the semantic analysis of the provided data. It is expected that the mentioned project will provide the overall description of both tasks together with determination of the models that are most suitable for the summarization and content tagging. This comparison aims to provide useful findings on the discrepancy between general and specialized models for accomplishing good quality text processing in real-world and practical context. |
Keywords | Text Summarization, Content Tagging, NLP, LLM, BERT, Fine-Tuning, Text Classification, Semantic Analysis, Model Comparison |
Field | Computer > Artificial Intelligence / Simulation / Virtual Reality |
Published In | Volume 7, Issue 3, May-June 2025 |
Published On | 2025-05-11 |
DOI | https://doi.org/10.36948/ijfmr.2025.v07i03.44507 |
Short DOI | https://doi.org/g9kfm6 |
Share this

E-ISSN 2582-2160

CrossRef DOI is assigned to each research paper published in our journal.
IJFMR DOI prefix is
10.36948/ijfmr
Downloads
All research papers published on this website are licensed under Creative Commons Attribution-ShareAlike 4.0 International License, and all rights belong to their respective authors/researchers.
