
International Journal For Multidisciplinary Research
E-ISSN: 2582-2160
•
Impact Factor: 9.24
A Widely Indexed Open Access Peer Reviewed Multidisciplinary Bi-monthly Scholarly International Journal
Home
Research Paper
Submit Research Paper
Publication Guidelines
Publication Charges
Upload Documents
Track Status / Pay Fees / Download Publication Certi.
Editors & Reviewers
View All
Join as a Reviewer
Get Membership Certificate
Current Issue
Publication Archive
Conference
Publishing Conf. with IJFMR
Upcoming Conference(s) ↓
WSMCDD-2025
GSMCDD-2025
AIMAR-2025
Conferences Published ↓
ICCE (2025)
RBS:RH-COVID-19 (2023)
ICMRS'23
PIPRDA-2023
Contact Us
Plagiarism is checked by the leading plagiarism checker
Call for Paper
Volume 7 Issue 4
July-August 2025
Indexing Partners

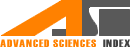
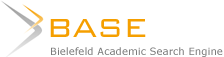



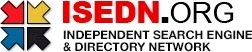

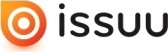

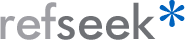
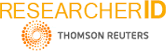
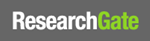
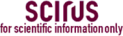
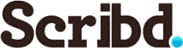


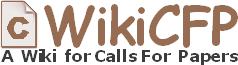

Automated Detection of Anomalies in Healthcare Data Using Machine Learning
Author(s) | Ravikanth Konda |
---|---|
Country | United States |
Abstract | The health care industry creates vast amounts of data each day, from electronic health records (EHRs) and lab test results to radiological images and outputs from wearable devices. This expanding reservoir of information offers an unprecedented potential for improving patient outcomes through data-driven decision making. Yet, the complexity and high dimensionality of healthcare data also pose significant risks, particularly in terms of errors, fraud, and missed clinical events. Manual anomaly reviewing and detection mechanisms tend to be inefficient, error-prone, and non-scalable. To circumvent these downsides, autonomous anomaly detection methods based on ML algorithms have increasingly become popular.This paper identifies how different algorithms of ML may be used in order to find anomalies in medical data efficiently. We examine both supervised and unsupervised algorithms like Support Vector Machines (SVM), Random Forests, Isolation Forests, Autoencoders, and k-Nearest Neighbors (k-NN). These models are compared in terms of their precision, recall, F1-score, and area under the ROC curve (AUC) on real-world datasets from hospital databases and publicly available healthcare repositories. The methodology section explains data preprocessing, model training, hyperparameter tuning, and validation techniques. Our experimental results show that ensemble models and deep learning architectures tend to outperform conventional methods in both accuracy and robustness, particularly in dealing with imbalanced datasets. In addition, we discuss the operational challenges in implementing these systems, such as data privacy issues, interpretability of sophisticated models, integration with hospital information systems in place, and regulatory compliance. The discussion presents solutions like differential privacy, explainability frameworks for models, and continuous learning systems to address these challenges. In conclusion, the results highlight the revolutionary potential of machine learning to improve anomaly detection, thus ensuring patient safety, optimizing healthcare provision, and enabling real-time clinical decision-making. |
Field | Engineering |
Published In | Volume 7, Issue 3, May-June 2025 |
Published On | 2025-05-14 |
DOI | https://doi.org/10.36948/ijfmr.2025.v07i03.45069 |
Short DOI | https://doi.org/g9kfw8 |
Share this

E-ISSN 2582-2160

CrossRef DOI is assigned to each research paper published in our journal.
IJFMR DOI prefix is
10.36948/ijfmr
Downloads
All research papers published on this website are licensed under Creative Commons Attribution-ShareAlike 4.0 International License, and all rights belong to their respective authors/researchers.
