
International Journal For Multidisciplinary Research
E-ISSN: 2582-2160
•
Impact Factor: 9.24
A Widely Indexed Open Access Peer Reviewed Multidisciplinary Bi-monthly Scholarly International Journal
Home
Research Paper
Submit Research Paper
Publication Guidelines
Publication Charges
Upload Documents
Track Status / Pay Fees / Download Publication Certi.
Editors & Reviewers
View All
Join as a Reviewer
Get Membership Certificate
Current Issue
Publication Archive
Conference
Publishing Conf. with IJFMR
Upcoming Conference(s) ↓
WSMCDD-2025
GSMCDD-2025
Conferences Published ↓
ICCE (2025)
RBS:RH-COVID-19 (2023)
ICMRS'23
PIPRDA-2023
Contact Us
Plagiarism is checked by the leading plagiarism checker
Call for Paper
Volume 7 Issue 3
May-June 2025
Indexing Partners

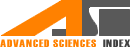
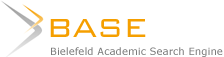



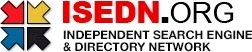

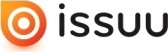

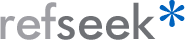
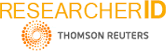
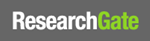
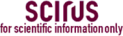
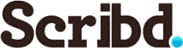


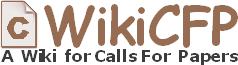

WebPedia 2.0: LLMs to Revolutionize Wikipedia Content Creation, Automating Knowledge Curation with Generative AI
Author(s) | Prof. Dr. JAYANTHI KANNAN M.K, Mr. Anurodh Pancholi, Mr. Priyaanshu Singh |
---|---|
Country | India |
Abstract | The WEBPEDIA (Synthesis of Topic-Oriented Role-based Multi-document Summarization) system is designed to produce structured summaries from multiple documents by organizing content according to topic and the roles of various stakeholders (e.g., government, media, public) involved in an event. The implementation begins with a Document Collection module that gathers relevant texts from different sources discussing the same event, ensuring a diverse, multi-perspective input. Next, the Topic Segmentation module uses techniques like TextRank and semantic clustering to break the content into coherent topic segments. The Role Identification module employs Named Entity Recognition (NER), dependency parsing, and rule-based methods to map entities to corresponding roles, such as identifying a political figure as part of the government perspective. Once roles are identified, the Role-based Content Selection module uses TF-IDF scores, semantic similarity, and topic-role alignment techniques to filter and select content that is most relevant to each identified role, removing redundant or off-topic text. For summary generation, WEBPEDIA combines template-based methods with neural text generation models such as BART or T5, which helps maintain fluency and coherence in the output while adhering to factual and logical flow. The final summary combines each role’s perspective into a comprehensive multi-document, multi-role narrative. For evaluation, the authors used datasets derived from real-world news coverage of complex events such as elections, pandemics, and protests. WEBPEDIA's performance was assessed using ROUGE metrics (ROUGE-1, ROUGE-2, ROUGE-L), as well as qualitative metrics like coverage and diversity to evaluate how well the summaries reflected all relevant roles and topics. Human evaluations further measured the fluency, coherence, and role accuracy of the summaries. The results showed that WEBPEDIA significantly outperforms traditional summarization baselines by generating summaries that are not only informative and factually consistent but also nuanced with multiple stakeholder perspectives, showcasing its effectiveness in complex information environment. |
Keywords | WebPedia 2.0, LLMs to Revolutionize Wikipedia, Content Creation Wikipedia, Automating Knowledge Curation, Generative AI, WEBpedia, Redefining Wikipedia, Crafting Wikipedia Articles with AI, Large Language Models. |
Field | Computer > Artificial Intelligence / Simulation / Virtual Reality |
Published In | Volume 7, Issue 2, March-April 2025 |
Published On | 2025-04-22 |
DOI | https://doi.org/10.36948/ijfmr.2025.v07i02.42262 |
Short DOI | https://doi.org/g9gdrd |
Share this

E-ISSN 2582-2160

CrossRef DOI is assigned to each research paper published in our journal.
IJFMR DOI prefix is
10.36948/ijfmr
Downloads
All research papers published on this website are licensed under Creative Commons Attribution-ShareAlike 4.0 International License, and all rights belong to their respective authors/researchers.
