
International Journal For Multidisciplinary Research
E-ISSN: 2582-2160
•
Impact Factor: 9.24
A Widely Indexed Open Access Peer Reviewed Multidisciplinary Bi-monthly Scholarly International Journal
Home
Research Paper
Submit Research Paper
Publication Guidelines
Publication Charges
Upload Documents
Track Status / Pay Fees / Download Publication Certi.
Editors & Reviewers
View All
Join as a Reviewer
Get Membership Certificate
Current Issue
Publication Archive
Conference
Publishing Conf. with IJFMR
Upcoming Conference(s) ↓
WSMCDD-2025
GSMCDD-2025
Conferences Published ↓
ICCE (2025)
RBS:RH-COVID-19 (2023)
ICMRS'23
PIPRDA-2023
Contact Us
Plagiarism is checked by the leading plagiarism checker
Call for Paper
Volume 7 Issue 3
May-June 2025
Indexing Partners

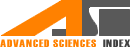
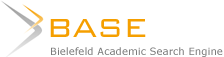



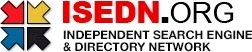

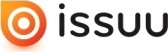

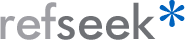
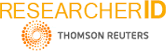
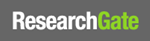
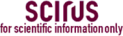
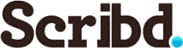


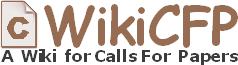

Leveraging Machine Learning and Ensemble Methods for Accurate Parkinson’s Disease Diagnosis: A Study on SMOTE-TomekLinks and SHAP Interpretability
Author(s) | Mr. Mohsin Amin Sofi |
---|---|
Country | India |
Abstract | Purpose: Parkinson’s disease (PD) is a progressive neurodegenerative disorder that affects millions globally, leading to significant impairments in both motor and non-motor functions. The early and accurate diagnosis of PD remains a critical challenge, as existing diagnostic methods often depend on the manifestation of advanced-stage symptoms. This study conducts a comprehensive comparative analysis of machine learning base models, evaluated both with and without the application of SMOTE-TomekLinks to address class imbalance. Additionally, the research integrates SHAP (SHapley Additive Explanations) analysis to ensure model interpretability and employs ensemble stacking techniques that combine the outputs of base models with two meta-models, XGBoost and AdaBoost, to enhance predictive accuracy and reliability. Methods: A dataset was collected from the UCI repository and preprocessed for normalization and feature selection. Six machine learning models, including Logistic Regression, Decision Tree, Random Forest, Support Vector Machines (SVM), K-Nearest Neighbors, and Naive Bayes, were trained and evaluated with and without SMOTE-TomekLinks. Ensemble techniques using XGBoost and AdaBoost were employed to enhance predictive accuracy. Model performance was assessed using metrics such as accuracy, F1-score, confusion matrices, and ROC-AUC. SHAP (SHapley Additive exPlanations) analysis was used to interpret feature importance. Results: SMOTE-TomekLinks significantly improved the performance of all models, with Random Forest achieving the highest accuracy (96.61%) among the base models. Ensemble techniques further enhanced performance, with XGBoost achieving the best results, including an accuracy of 98.30%, an F1-score of 0.98 for both classes, and an ROC-AUC of 0.98. SHAP analysis identified key features such as spread1, spread2, PPE, and MDVP:Fo(Hz) as critical for classification. Conclusion: The study demonstrates the transformative potential of combining advanced preprocessing, class-balancing techniques, and ensemble methods in diagnosing Parkinson’s disease. The findings emphasize the importance of addressing class imbalance to achieve reliable and interpretable diagnostic tools, bridging the gap between computational approaches and clinical applications hence, improving patient outcomes. |
Keywords | Parkinson’s Disease (PD), Machine Learning, Biomedical Voice Measurements , Synthetic Minority Oversampling Technique (SMOTE)-TomekLinks, SHAP (SHapley Additive exPlanations), XGBoost(Extreme Gradient Boosting), AdaBoost(Adaptive Boosting ) |
Field | Computer > Artificial Intelligence / Simulation / Virtual Reality |
Published In | Volume 7, Issue 2, March-April 2025 |
Published On | 2025-04-22 |
DOI | https://doi.org/10.36948/ijfmr.2025.v07i02.42372 |
Short DOI | https://doi.org/g9gdrb |
Share this

E-ISSN 2582-2160

CrossRef DOI is assigned to each research paper published in our journal.
IJFMR DOI prefix is
10.36948/ijfmr
Downloads
All research papers published on this website are licensed under Creative Commons Attribution-ShareAlike 4.0 International License, and all rights belong to their respective authors/researchers.
